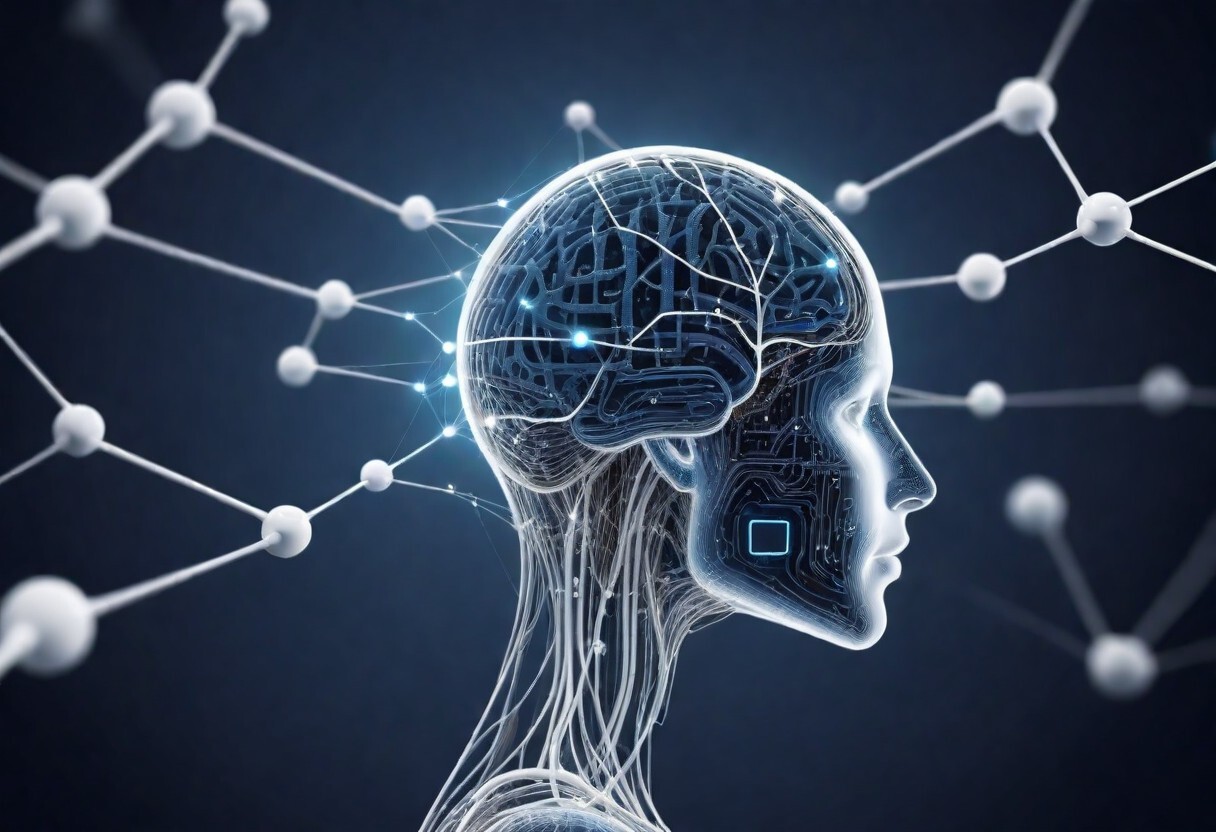
Machine learning models have become a hot topic in recent years, but what exactly are they? Simply put, machine learning models are algorithms that allow computers to learn from data and make predictions or decisions without being explicitly programmed. These models can be found in everyday applications like voice assistants, recommendation systems, and even self-driving cars. But how do they work? They use patterns and relationships within data to improve their performance over time. Curious about the magic behind these models? Let's dive into 25 fascinating facts that will help you understand the power and potential of machine learning models.
What is Machine Learning?
Machine learning (ML) is a branch of artificial intelligence where computers learn from data to make decisions or predictions. These models can identify patterns, improve over time, and adapt to new information.
- Machine learning models are algorithms that can learn from and make predictions on data.
- Supervised learning involves training a model on labeled data, where the correct output is known.
- Unsupervised learning uses data without labeled responses, finding hidden patterns or intrinsic structures.
- Reinforcement learning teaches models to make sequences of decisions by rewarding desired behaviors.
- Deep learning is a subset of ML that uses neural networks with many layers to analyze complex data.
Types of Machine Learning Models
Different types of models serve various purposes, from simple linear regressions to complex neural networks.
- Linear regression predicts continuous outcomes based on input features.
- Logistic regression is used for binary classification problems.
- Decision trees split data into branches to make predictions based on feature values.
- Random forests combine multiple decision trees to improve accuracy.
- Support vector machines (SVMs) find the best boundary to separate different classes.
Applications of Machine Learning
Machine learning models are used in numerous fields, transforming industries and everyday life.
- Healthcare uses ML for diagnosing diseases, predicting patient outcomes, and personalizing treatments.
- Finance employs ML for fraud detection, risk management, and algorithmic trading.
- Retail benefits from ML through personalized recommendations, inventory management, and customer segmentation.
- Transportation relies on ML for route optimization, autonomous driving, and predictive maintenance.
- Entertainment platforms use ML to recommend movies, music, and other content based on user preferences.
Challenges in Machine Learning
Despite its potential, machine learning faces several hurdles that researchers and practitioners must overcome.
- Data quality is crucial, as poor data can lead to inaccurate models.
- Overfitting occurs when a model learns the training data too well, failing to generalize to new data.
- Bias and fairness are concerns, as models can perpetuate existing biases in the data.
- Interpretability is important, as complex models like deep learning can be difficult to understand.
- Scalability is necessary for handling large datasets and real-time predictions.
Future of Machine Learning
The future of machine learning holds exciting possibilities, with advancements in technology and new applications emerging.
- Quantum computing could revolutionize ML by solving problems that are currently infeasible.
- Edge computing brings ML closer to data sources, reducing latency and improving efficiency.
- Automated machine learning (AutoML) aims to make ML accessible to non-experts by automating model selection and tuning.
- Explainable AI (XAI) focuses on making ML models more transparent and understandable.
- Ethical AI emphasizes the importance of developing ML systems that are fair, accountable, and aligned with human values.
Final Thoughts on Machine Learning Models
Machine learning models have transformed how we approach problems, making tasks like image recognition, language translation, and even medical diagnoses more efficient. These models learn from data, improving their accuracy over time. They’re not just for tech giants; businesses of all sizes can harness their power. However, they require vast amounts of data and computational resources. Ethical considerations, like bias and privacy, are crucial when developing and deploying these models. Understanding the basics of machine learning can open doors to countless opportunities. Whether you're a student, professional, or hobbyist, diving into this field can be incredibly rewarding. Keep exploring, stay curious, and remember that the world of machine learning is vast and ever-evolving. The future holds even more exciting advancements, so stay tuned and keep learning.
Was this page helpful?
Our commitment to delivering trustworthy and engaging content is at the heart of what we do. Each fact on our site is contributed by real users like you, bringing a wealth of diverse insights and information. To ensure the highest standards of accuracy and reliability, our dedicated editors meticulously review each submission. This process guarantees that the facts we share are not only fascinating but also credible. Trust in our commitment to quality and authenticity as you explore and learn with us.