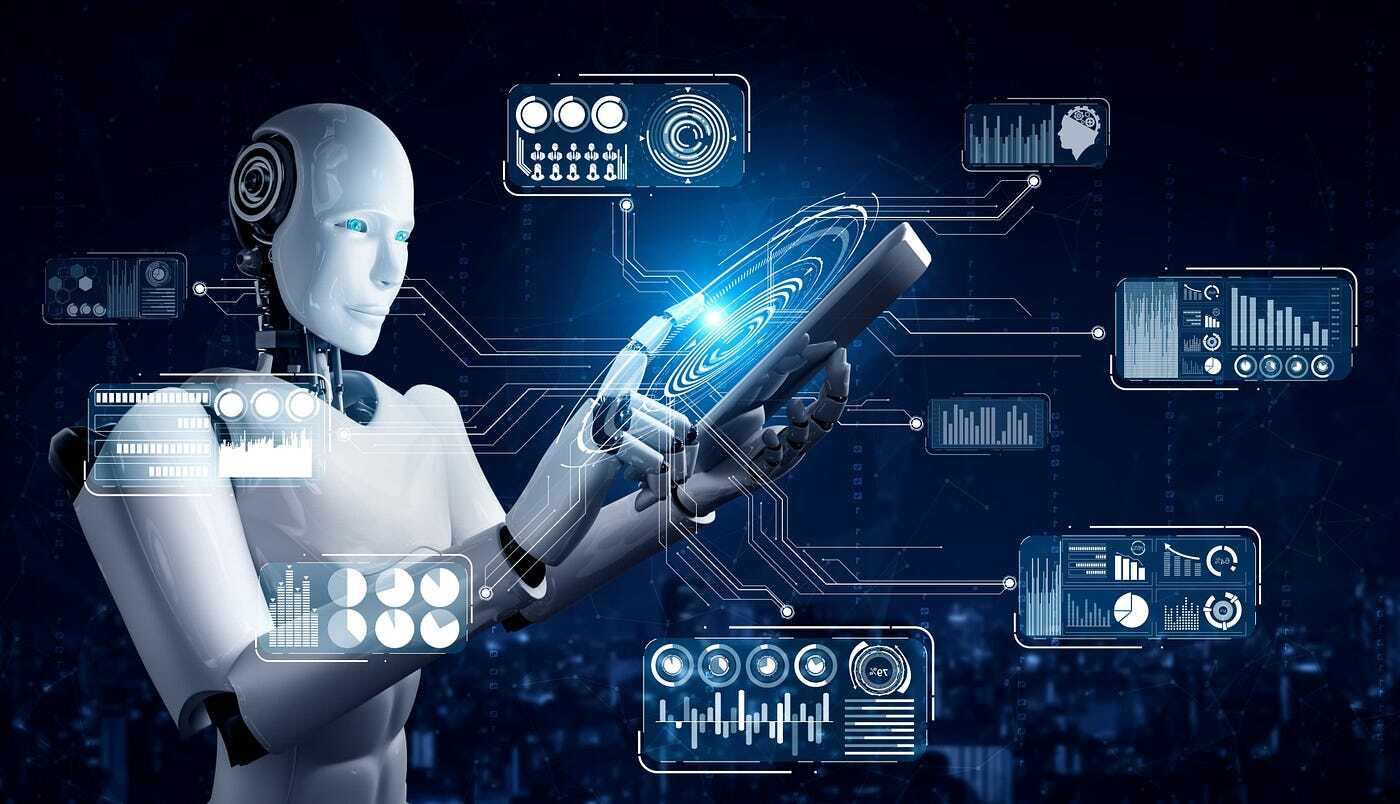
Reinforcement learning, a dynamic branch of artificial intelligence, teaches machines to make decisions by rewarding desired behaviors and penalizing errors. Imagine teaching a dog new tricks; positive reinforcement helps it learn what actions lead to treats. Similarly, reinforcement learning algorithms learn optimal actions through trial and error, aiming to maximize rewards over time. This approach has powered breakthroughs in autonomous vehicles, game-playing AI, and personalized recommendations, showcasing its versatility and potential. With its roots in psychology and computer science, reinforcement learning stands as a testament to the power of learning from interaction, continuously evolving as researchers uncover new applications and techniques. Reinforcement learning not only revolutionizes how machines learn but also offers insights into human learning processes, bridging gaps between technology and natural intelligence.
What is Reinforcement Learning?
Reinforcement learning is a type of machine learning where an agent learns to make decisions by taking actions in an environment to achieve some goals. Unlike traditional forms of machine learning, where models are trained on a fixed dataset, reinforcement learning models learn from trial and error, receiving feedback from their own actions and experiences.
- Reinforcement learning is inspired by behavioral psychology and involves learning to map situations to actions with the goal of maximizing a numerical reward signal.
Key Components of Reinforcement Learning
At its core, reinforcement learning consists of several key components that interact with each other to enable machines or software agents to learn from their environment.
- An agent is the learner or decision-maker.
- The environment includes everything the agent interacts with.
- Actions are what the agent can do.
- Rewards are feedback from the environment based on the actions taken by the agent.
How Does Reinforcement Learning Work?
The process of reinforcement learning involves the agent taking actions in an environment, which leads to changes in the state of the environment and the agent receiving rewards. These rewards guide the agent in learning which actions are beneficial.
- The agent uses a policy to decide its actions, which can be seen as a strategy that takes into account the current state and the expected future rewards.
- Value functions estimate how good it is for the agent to be in a given state or how good it is to perform a certain action in a state.
Applications of Reinforcement Learning
Reinforcement learning has a wide range of applications, from gaming to robotics, and its versatility is one of its most compelling attributes.
- In video games, reinforcement learning can be used to develop AI that adapts to the player’s strategy.
- Autonomous vehicles use reinforcement learning to make decisions in real-time, such as when to brake or accelerate.
- Robotics applications often involve reinforcement learning to enable robots to learn how to perform tasks through trial and error.
Challenges in Reinforcement Learning
While reinforcement learning holds great promise, it also faces several challenges that researchers and practitioners are actively working to overcome.
- One major challenge is the balance between exploration and exploitation, where the agent must try new actions to discover their effects while also using known actions that yield high rewards.
- Sparse rewards can make it difficult for the agent to learn, as feedback on actions may not be immediate or frequent.
- Scalability is another challenge, as reinforcement learning models can require significant computational resources, especially for complex environments.
Future of Reinforcement Learning
The future of reinforcement learning is bright, with ongoing research and advancements opening up new possibilities and applications.
- Transfer learning and meta-learning are areas of research that aim to enable agents to apply knowledge learned in one task to other, similar tasks.
- Multi-agent reinforcement learning explores how multiple agents can learn together or compete within the same environment, which could revolutionize areas like economics, traffic systems, and more.
Reinforcement Learning in Everyday Life
You might not realize it, but reinforcement learning is already part of our everyday lives, influencing various technologies and services we use.
- Personalized recommendations on platforms like Netflix or YouTube use reinforcement learning to tailor content to individual user preferences.
- Smart home devices learn from your habits and preferences to optimize energy use or adjust settings automatically.
Reinforcement Learning vs. Other Machine Learning Types
Understanding how reinforcement learning differs from other types of machine learning can highlight its unique advantages and challenges.
- Unlike supervised learning, where models learn from labeled data, reinforcement learning learns from the consequences of actions.
- Unsupervised learning involves finding patterns in data, but reinforcement learning is focused on learning a policy to maximize rewards.
Key Takeaways
Reinforcement learning is a dynamic and rapidly evolving field with the potential to revolutionize how machines learn and interact with the world.
- As technology advances, reinforcement learning will likely become even more integrated into our daily lives, powering innovations that we can only begin to imagine.
A Final Glimpse at Reinforcement Learning
Reinforcement learning, a cornerstone of modern AI, has shown us how machines can learn from their actions, much like humans do. From mastering complex games to driving autonomous vehicles, this technology's impact is profound and far-reaching. As we've seen, its applications span various fields, making everyday tasks more efficient and opening doors to new possibilities. Whether it's healthcare, finance, robotics, or entertainment, reinforcement learning is reshaping industries, making processes smarter and outcomes better. Remember, the journey of understanding and applying this dynamic field is ongoing. Staying curious and informed about its developments will undoubtedly pay off, as the future of technology continues to evolve around these intelligent systems. So, keep exploring, and let's see where this fascinating branch of AI takes us next.
Was this page helpful?
Our commitment to delivering trustworthy and engaging content is at the heart of what we do. Each fact on our site is contributed by real users like you, bringing a wealth of diverse insights and information. To ensure the highest standards of accuracy and reliability, our dedicated editors meticulously review each submission. This process guarantees that the facts we share are not only fascinating but also credible. Trust in our commitment to quality and authenticity as you explore and learn with us.