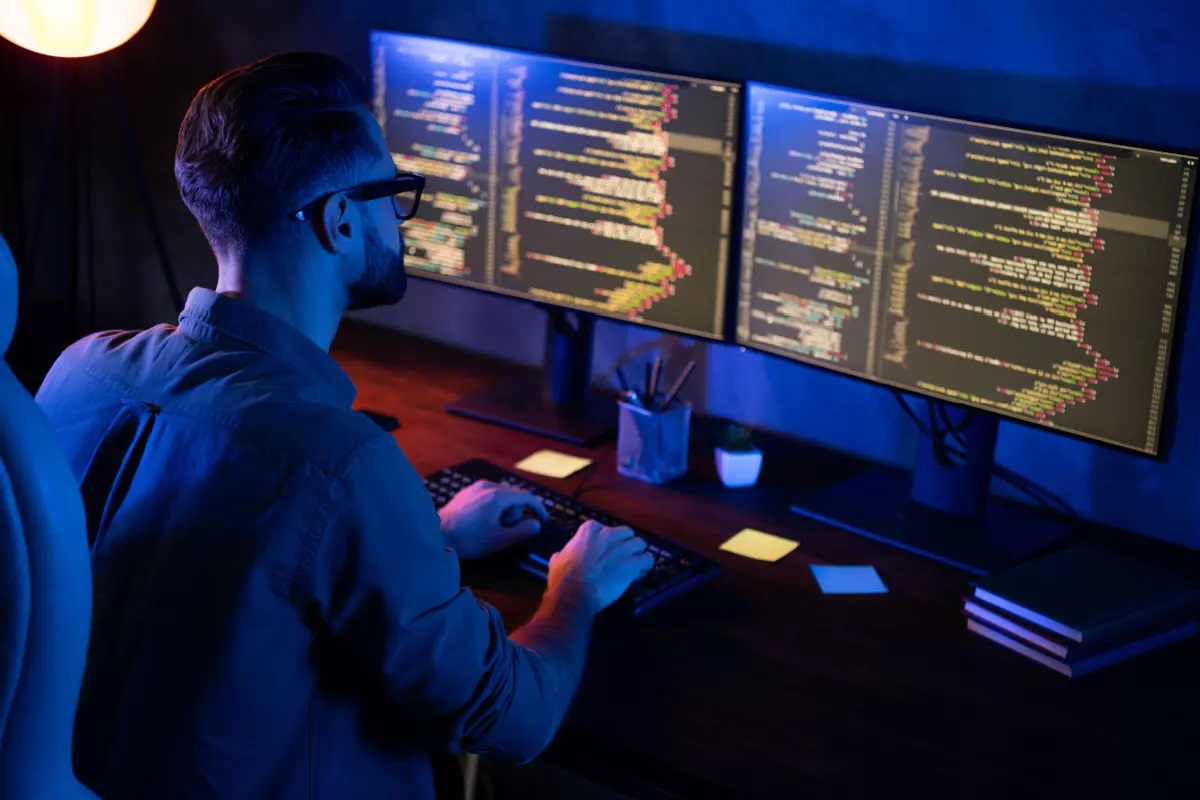
Model monitoring is crucial for ensuring machine learning models perform well over time. But what exactly does it involve? Model monitoring tracks a model's performance, identifying issues like data drift, concept drift, and unexpected behaviors. This process helps maintain accuracy and reliability, ensuring predictions remain relevant. Without it, models can degrade, leading to poor decisions. Model monitoring involves various techniques and tools, each designed to catch different types of problems. From tracking metrics to setting up alerts, it’s a comprehensive approach to keeping models in check. Ready to dive deeper? Here are 17 facts about model monitoring that will help you understand its importance and how it works.
What is Model Monitoring?
Model monitoring is the process of continuously checking the performance and behavior of machine learning models in production. It ensures models remain accurate and reliable over time.
-
Model Drift: Over time, the data a model was trained on may change. This phenomenon, known as model drift, can lead to decreased performance.
-
Data Quality: Monitoring helps identify issues with data quality, such as missing values or outliers, which can impact model accuracy.
-
Performance Metrics: Key metrics like accuracy, precision, recall, and F1 score are tracked to gauge model performance.
-
Real-Time Monitoring: Some systems provide real-time monitoring, allowing for immediate detection and correction of issues.
Why is Model Monitoring Important?
Model monitoring is crucial for maintaining the reliability and effectiveness of machine learning models. It helps in identifying and addressing potential issues before they impact business decisions.
-
Early Detection: Early detection of performance degradation can prevent significant business losses.
-
Compliance: Ensures models comply with regulatory requirements, which is essential in industries like finance and healthcare.
-
User Trust: Maintaining high model performance builds trust among users and stakeholders.
-
Resource Optimization: Helps in optimizing computational resources by identifying inefficient models.
Techniques for Model Monitoring
Various techniques are employed to monitor models effectively. These techniques help in identifying issues and maintaining model performance.
-
Statistical Tests: Statistical tests like the Kolmogorov-Smirnov test can detect changes in data distribution.
-
Visualization Tools: Tools like dashboards and visualizations help in understanding model performance at a glance.
-
Alert Systems: Automated alert systems notify data scientists of any anomalies or performance drops.
-
Shadow Deployment: Running a new model alongside the old one to compare performance without affecting the end-users.
Challenges in Model Monitoring
Despite its importance, model monitoring comes with its own set of challenges. Understanding these challenges can help in better implementation.
-
Scalability: Monitoring multiple models in real-time can be resource-intensive and complex.
-
Data Privacy: Ensuring data privacy while monitoring models is a significant concern, especially with sensitive data.
-
Integration: Integrating monitoring tools with existing systems can be challenging and may require custom solutions.
-
Interpretability: Making sense of the monitoring data and translating it into actionable insights can be difficult.
Future of Model Monitoring
The future of model monitoring looks promising with advancements in technology and methodologies. These advancements aim to make monitoring more efficient and effective.
- AI-Powered Monitoring: Using AI to monitor other AI models can lead to more accurate and efficient monitoring processes.
Final Thoughts on Model Monitoring
Model monitoring is crucial for maintaining the accuracy and reliability of machine learning models. Regular checks help catch errors early, ensuring models perform as expected. Ignoring this can lead to flawed predictions and poor decisions. By keeping an eye on metrics like accuracy, precision, and recall, you can spot issues before they become big problems.
Automated tools make this easier, providing real-time insights and alerts. They save time and reduce human error. Remember, a well-monitored model is a trustworthy model. It’s not just about building a model; it’s about maintaining it too.
So, keep your models in check, use the right tools, and stay proactive. This way, you’ll ensure your machine learning efforts are always on point, delivering the best results possible. Happy monitoring!
Was this page helpful?
Our commitment to delivering trustworthy and engaging content is at the heart of what we do. Each fact on our site is contributed by real users like you, bringing a wealth of diverse insights and information. To ensure the highest standards of accuracy and reliability, our dedicated editors meticulously review each submission. This process guarantees that the facts we share are not only fascinating but also credible. Trust in our commitment to quality and authenticity as you explore and learn with us.