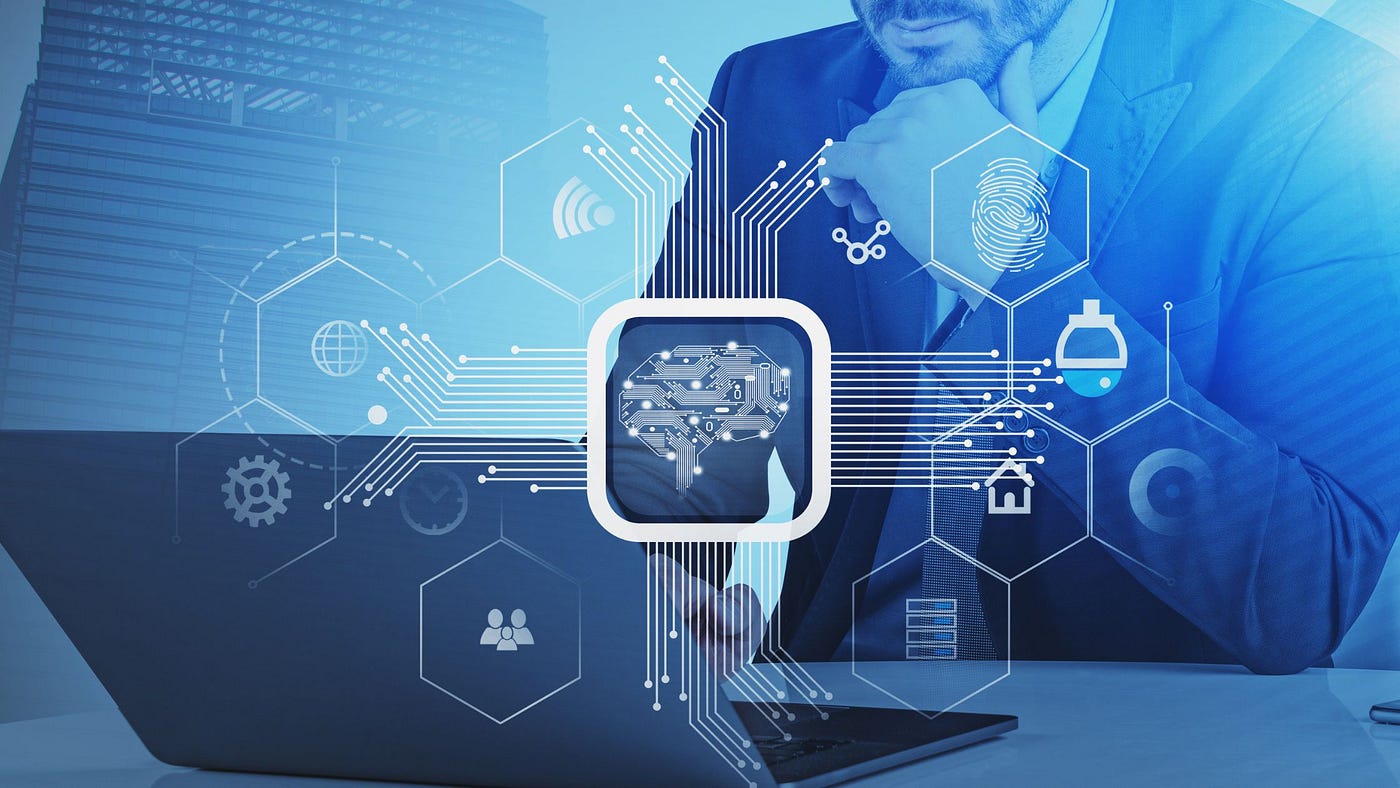
MLOps, a portmanteau of "machine learning" and "operations," is a burgeoning field that combines the principles of DevOps with machine learning. This innovative approach aims to streamline the deployment, monitoring, and management of machine learning models in production. As organizations increasingly leverage machine learning to drive business insights and enhance decision-making processes, the need for efficient MLOps practices becomes paramount.
In this article, we'll delve into 11 intriguing facts about MLOps, shedding light on its significance, evolution, and impact on the tech and sciences landscape. From its pivotal role in accelerating model deployment to its integration with data engineering and automation, MLOps is reshaping the way machine learning projects are executed and maintained. Join us as we unravel the complexities and opportunities within the realm of MLOps, offering valuable insights into this transformative discipline.
Key Takeaways:
- MLOps combines machine learning and DevOps to make it easier for companies to use and manage machine learning models. It helps teams work together, automates tasks, and ensures models perform well.
- MLOps helps organizations deploy and manage machine learning models more efficiently, leading to faster innovation and better collaboration between different teams. It makes sure models work well and follow the rules.
MLOps is an Integration of "Machine Learning" and "DevOps"
MLOps, a portmanteau of "Machine Learning" and "DevOps," represents the convergence of these two domains to streamline the deployment and management of machine learning models in production. This fusion enables organizations to efficiently develop, deploy, and monitor machine learning models, ensuring seamless integration with existing systems.
MLOps Enhances Collaboration Between Data Scientists and Operations Teams
MLOps facilitates a cohesive workflow by fostering collaboration between data scientists and operations teams. This synergy ensures that machine learning models are effectively operationalized, leading to improved efficiency and productivity across the organization.
MLOps Automates Model Deployment and Monitoring
One of the key benefits of MLOps is its ability to automate the deployment and monitoring of machine learning models. By leveraging MLOps practices, organizations can establish robust pipelines for model deployment and monitoring, thereby reducing manual intervention and enhancing scalability.
MLOps Ensures Model Performance and Reliability
Through MLOps, organizations can uphold the performance and reliability of machine learning models in production environments. This is achieved by implementing continuous monitoring and feedback loops, enabling timely adjustments to maintain optimal model performance.
MLOps Empowers Iterative Model Development and Improvement
MLOps empowers organizations to embrace iterative model development and improvement. By integrating feedback loops and version control, MLOps enables data scientists to continuously enhance machine learning models, fostering innovation and agility.
MLOps Enhances Model Governance and Compliance
With MLOps, organizations can establish robust governance and compliance frameworks for machine learning models. This ensures adherence to regulatory standards and internal policies, mitigating risks associated with model deployment and usage.
MLOps Enables Scalable and Reproducible Model Deployment
MLOps paves the way for scalable and reproducible model deployment, allowing organizations to efficiently deploy models across diverse environments while maintaining consistency and reliability.
MLOps Facilitates Efficient Model Versioning and Tracking
Through MLOps practices, organizations can effectively manage model versioning and tracking, enabling seamless collaboration and ensuring transparency throughout the model development lifecycle.
MLOps Optimizes Resource Utilization for Model Training and Inference
MLOps optimizes resource utilization for model training and inference, leading to improved efficiency and cost-effectiveness in machine learning operations.
MLOps Drives Business Value Through Accelerated Model Deployment
By embracing MLOps, organizations can drive significant business value through accelerated model deployment, enabling faster time-to-market for innovative machine learning solutions.
MLOps Empowers Organizations to Harness the Full Potential of Machine Learning
MLOps empowers organizations to harness the full potential of machine learning by bridging the gap between development and operations, fostering a culture of collaboration, innovation, and continuous improvement.
MLOps represents a pivotal advancement in the realm of machine learning, offering a holistic approach to operationalizing and managing machine learning models. By integrating the principles of DevOps with the intricacies of machine learning, MLOps enables organizations to streamline model deployment, enhance collaboration, and drive business value through accelerated innovation. This convergence of disciplines underscores the significance of MLOps in empowering organizations to leverage machine learning effectively, thereby shaping a future where intelligent systems seamlessly integrate into diverse operational landscapes.
Conclusion
In conclusion, MLOps is revolutionizing the way organizations develop, deploy, and manage machine learning models. By integrating DevOps principles with machine learning workflows, MLOps streamlines the entire ML lifecycle, from data preparation to model deployment and monitoring. This approach enhances collaboration between data scientists, engineers, and operations teams, leading to faster model iteration and improved deployment reliability. As businesses increasingly rely on AI and ML, embracing MLOps practices becomes crucial for achieving scalable, efficient, and reliable machine learning operations.
FAQs
What are the key components of MLOps?MLOps encompasses various components, including version control for ML models, continuous integration and deployment (CI/CD) pipelines, model monitoring, and automated testing frameworks. These components ensure seamless collaboration and efficient management of machine learning workflows.
How does MLOps differ from DevOps?While DevOps focuses on the software development lifecycle, MLOps specifically addresses the unique challenges of deploying and managing machine learning models. MLOps integrates ML-specific tools and practices into the DevOps framework, catering to the complexities of machine learning operations.
Was this page helpful?
Our commitment to delivering trustworthy and engaging content is at the heart of what we do. Each fact on our site is contributed by real users like you, bringing a wealth of diverse insights and information. To ensure the highest standards of accuracy and reliability, our dedicated editors meticulously review each submission. This process guarantees that the facts we share are not only fascinating but also credible. Trust in our commitment to quality and authenticity as you explore and learn with us.