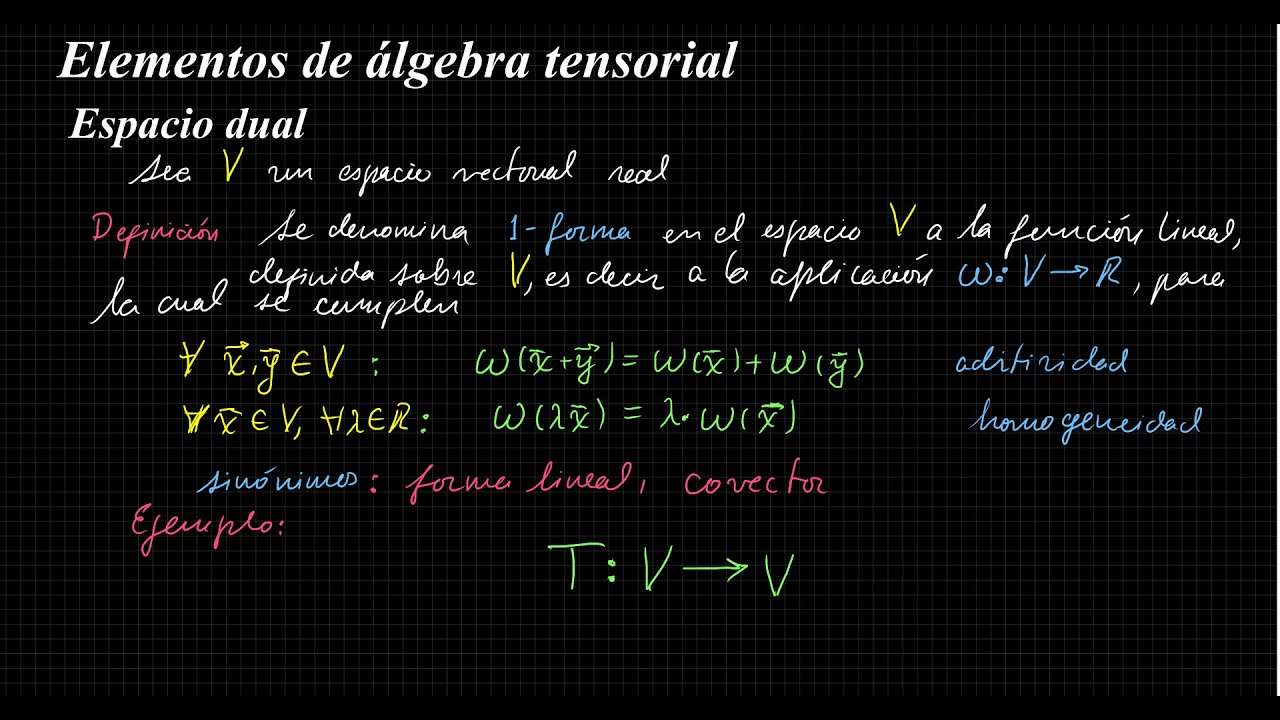
What is Tensorial? Tensorial is a mathematical concept used in various fields like physics, engineering, and computer science. It involves tensors, which are multi-dimensional arrays of numbers that generalize scalars, vectors, and matrices. Tensors can represent complex data structures and relationships. They are crucial in areas such as machine learning, especially in deep learning frameworks like TensorFlow. Understanding tensors helps in solving intricate problems related to data manipulation, transformations, and computations. Tensorial concepts are foundational for modern technologies like artificial intelligence and scientific simulations. In essence, Tensorial provides a robust framework for handling multi-dimensional data efficiently.
What is Tensorial?
Tensorial is a mathematical concept used in various fields like physics, engineering, and computer science. It helps in understanding complex structures and relationships. Here are some fascinating facts about tensorial.
- 01
Tensors are Generalizations: Tensors generalize scalars, vectors, and matrices. Scalars are zero-order tensors, vectors are first-order, and matrices are second-order.
- 02
Used in Physics: In physics, tensors describe physical properties like stress, strain, and moment of inertia.
- 03
Einstein's Theory: Albert Einstein used tensors in his theory of general relativity to describe the curvature of spacetime.
- 04
Tensor Notation: Tensor notation simplifies equations involving multiple dimensions, making them easier to manipulate.
- 05
Rank of a Tensor: The rank of a tensor indicates the number of dimensions. A rank-3 tensor has three dimensions.
- 06
Tensor Operations: Common tensor operations include addition, subtraction, and multiplication, similar to matrix operations.
Applications in Machine Learning
Tensorial concepts are crucial in machine learning and artificial intelligence. They help in handling large datasets and complex computations.
- 07
TensorFlow: TensorFlow, an open-source library by Google, uses tensors for numerical computations and machine learning models.
- 08
Neural Networks: Tensors represent data and parameters in neural networks, facilitating efficient computation.
- 09
Deep Learning: In deep learning, tensors handle multi-dimensional data like images and videos.
- 10
Data Representation: Tensors efficiently represent high-dimensional data, making them ideal for machine learning tasks.
- 11
Tensor Decomposition: Tensor decomposition techniques reduce the complexity of data, improving computational efficiency.
Tensorial in Engineering
Engineering fields use tensorial concepts to solve complex problems involving multiple dimensions and variables.
- 12
Finite Element Analysis: Engineers use tensors in finite element analysis to study stress and strain in materials.
- 13
Fluid Dynamics: Tensors describe the flow of fluids, helping engineers design efficient systems.
- 14
Electromagnetism: In electromagnetism, tensors represent electric and magnetic fields.
- 15
Structural Analysis: Tensors help in analyzing the structural integrity of buildings and bridges.
Mathematical Properties
Understanding the mathematical properties of tensors is essential for their application in various fields.
- 16
Symmetric Tensors: Symmetric tensors remain unchanged when indices are swapped, simplifying calculations.
- 17
Antisymmetric Tensors: Antisymmetric tensors change sign when indices are swapped, useful in physics.
- 18
Tensor Product: The tensor product combines two tensors to form a higher-order tensor.
- 19
Contraction: Tensor contraction reduces the order of a tensor by summing over specific indices.
- 20
Eigenvalues and Eigenvectors: Tensors have eigenvalues and eigenvectors, similar to matrices, used in various applications.
Historical Context
The development of tensorial concepts has a rich history, contributing to advancements in science and mathematics.
- 21
Riemannian Geometry: Bernhard Riemann developed Riemannian geometry, which uses tensors to describe curved spaces.
- 22
Ricci Tensor: Gregorio Ricci-Curbastro and Tullio Levi-Civita developed the Ricci tensor, fundamental in general relativity.
- 23
Tensor Calculus: Tensor calculus, developed in the 19th century, provides tools for manipulating tensors.
- 24
Einstein Summation Convention: Einstein introduced a notation to simplify tensor equations, known as the Einstein summation convention.
Tensorial in Computer Graphics
Computer graphics use tensorial concepts to create realistic images and animations.
- 25
3D Transformations: Tensors represent 3D transformations, enabling realistic rendering of objects.
- 26
Lighting Models: Tensors help simulate lighting effects, enhancing the realism of computer-generated images.
- 27
Texture Mapping: In texture mapping, tensors map textures onto 3D models, improving visual quality.
- 28
Animation: Tensors describe the motion of objects in animations, ensuring smooth transitions.
Future of Tensorial
The future holds exciting possibilities for tensorial applications, driven by advancements in technology and research.
- 29
Quantum Computing: Tensors may play a role in quantum computing, representing quantum states and operations.
- 30
Big Data: Tensors handle large datasets efficiently, crucial for big data analytics.
- 31
Robotics: In robotics, tensors model the dynamics of robots, improving control and performance.
- 32
Medical Imaging: Tensors enhance medical imaging techniques, providing detailed insights into the human body.
- 33
Climate Modeling: Tensors help in climate modeling, predicting weather patterns and climate change impacts.
The Final Word on Tensorial
Tensorial's got some pretty cool facts, right? From its roots in mathematics to its applications in machine learning, it’s clear this concept is more than just a fancy term. Tensorial structures help in understanding complex data, making predictions, and even in creating AI models. They’re like the unsung heroes behind many tech advancements we see today.
Understanding tensorial concepts can give you a leg up in fields like data science, physics, and engineering. Whether you're a student, a professional, or just a curious mind, knowing about tensors can open up new ways of thinking and problem-solving.
So next time you hear the word "tensorial," you'll know it's not just jargon. It's a key player in the world of science and technology. Keep exploring, keep learning, and who knows? Maybe you'll uncover the next big thing using tensors!
Was this page helpful?
Our commitment to delivering trustworthy and engaging content is at the heart of what we do. Each fact on our site is contributed by real users like you, bringing a wealth of diverse insights and information. To ensure the highest standards of accuracy and reliability, our dedicated editors meticulously review each submission. This process guarantees that the facts we share are not only fascinating but also credible. Trust in our commitment to quality and authenticity as you explore and learn with us.