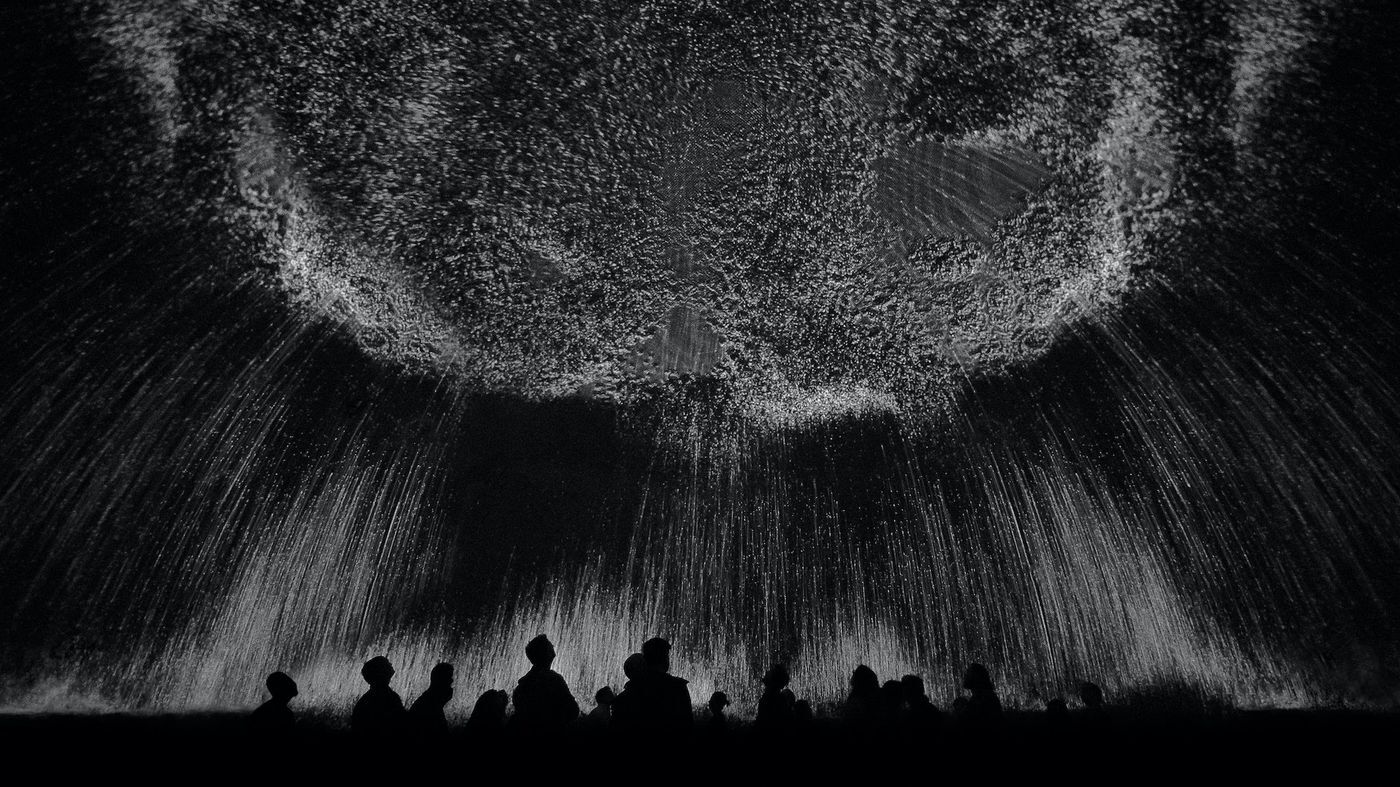
Latent space might sound like something out of a sci-fi movie, but it's a crucial concept in machine learning and artificial intelligence. What is latent space? Latent space is a multi-dimensional space where complex data is simplified and represented in a way that makes it easier for algorithms to understand and process. Imagine trying to describe a picture to someone without showing it; you’d break it down into simpler parts. That's what latent space does with data. It helps AI systems recognize patterns, make predictions, and even create new content. Curious about how it all works? Let's dive into 19 fascinating facts about latent space!
What is Latent Space?
Latent space is a concept from machine learning and artificial intelligence. It represents a compressed version of data, capturing essential features while discarding noise. This space is crucial for tasks like image generation, natural language processing, and more.
-
Latent space is a lower-dimensional representation of data. It simplifies complex data into essential features, making it easier to analyze and manipulate.
-
Autoencoders often use latent space. These neural networks compress data into a latent space and then reconstruct it, ensuring important features are retained.
-
Generative models like GANs (Generative Adversarial Networks) rely on latent space. They generate new data by sampling from this space, creating realistic images, text, or audio.
How Latent Space Works
Understanding how latent space functions can demystify many AI processes. Here's a closer look at its mechanics.
-
Dimensionality reduction is key. Techniques like PCA (Principal Component Analysis) reduce data dimensions, making latent space more manageable.
-
Feature extraction happens in latent space. It captures the most informative aspects of data, enabling better performance in tasks like classification or clustering.
-
Interpolation within latent space allows smooth transitions between data points. This is useful in generating variations of images or other data types.
Applications of Latent Space
Latent space has numerous applications across various fields. Let's explore some of the most impactful ones.
-
Image generation benefits greatly. Models like GANs create new images by sampling from latent space, producing realistic and diverse outputs.
-
Natural language processing (NLP) uses latent space for tasks like text generation and translation. It captures semantic meaning, improving the quality of generated text.
-
Anomaly detection leverages latent space. By understanding normal data patterns, it can identify outliers or unusual behavior effectively.
Latent Space in Deep Learning
Deep learning models often utilize latent space for various tasks. Here's how it integrates into these advanced systems.
-
Variational Autoencoders (VAEs) use latent space to generate new data. They introduce randomness, making the generated data more diverse and realistic.
-
Style transfer in images employs latent space. It separates content and style, allowing the combination of different artistic elements.
-
Reinforcement learning can benefit from latent space. It helps in state representation, making it easier for agents to learn and make decisions.
Challenges and Limitations
While latent space offers many advantages, it also comes with challenges. Understanding these can help in better utilizing this concept.
-
Interpretability is a major issue. Latent space representations are often abstract, making it hard to understand what each dimension represents.
-
Overfitting can occur. If the latent space is too complex, the model might capture noise instead of meaningful patterns.
-
Scalability poses a problem. As data grows, managing and navigating latent space becomes increasingly difficult.
Future of Latent Space
The future holds exciting possibilities for latent space. Innovations and advancements continue to push the boundaries of what’s possible.
-
Quantum computing might revolutionize latent space. It could handle larger and more complex data, making latent space more powerful.
-
Hybrid models combining latent space with other techniques could enhance performance. This could lead to more robust and versatile AI systems.
-
Ethical considerations will become crucial. Ensuring latent space models are fair and unbiased will be a key focus.
-
Real-time applications will expand. Latent space could enable faster and more efficient real-time data processing, benefiting various industries.
The Final Word on Latent Space
Latent space is a fascinating concept in machine learning and artificial intelligence. It’s where data gets transformed into a compressed, abstract representation, making it easier for models to understand and work with. This space helps in generating new data, improving predictions, and even creating art. Understanding latent space can give you a better grasp of how AI models operate and why they’re so powerful.
Whether you’re a tech enthusiast or just curious about AI, knowing about latent space can open up new perspectives. It’s a key piece of the puzzle in the world of machine learning. So next time you hear about AI creating something amazing, remember, latent space is likely playing a big role. Keep exploring, stay curious, and who knows what other fascinating facts you’ll uncover!
Was this page helpful?
Our commitment to delivering trustworthy and engaging content is at the heart of what we do. Each fact on our site is contributed by real users like you, bringing a wealth of diverse insights and information. To ensure the highest standards of accuracy and reliability, our dedicated editors meticulously review each submission. This process guarantees that the facts we share are not only fascinating but also credible. Trust in our commitment to quality and authenticity as you explore and learn with us.