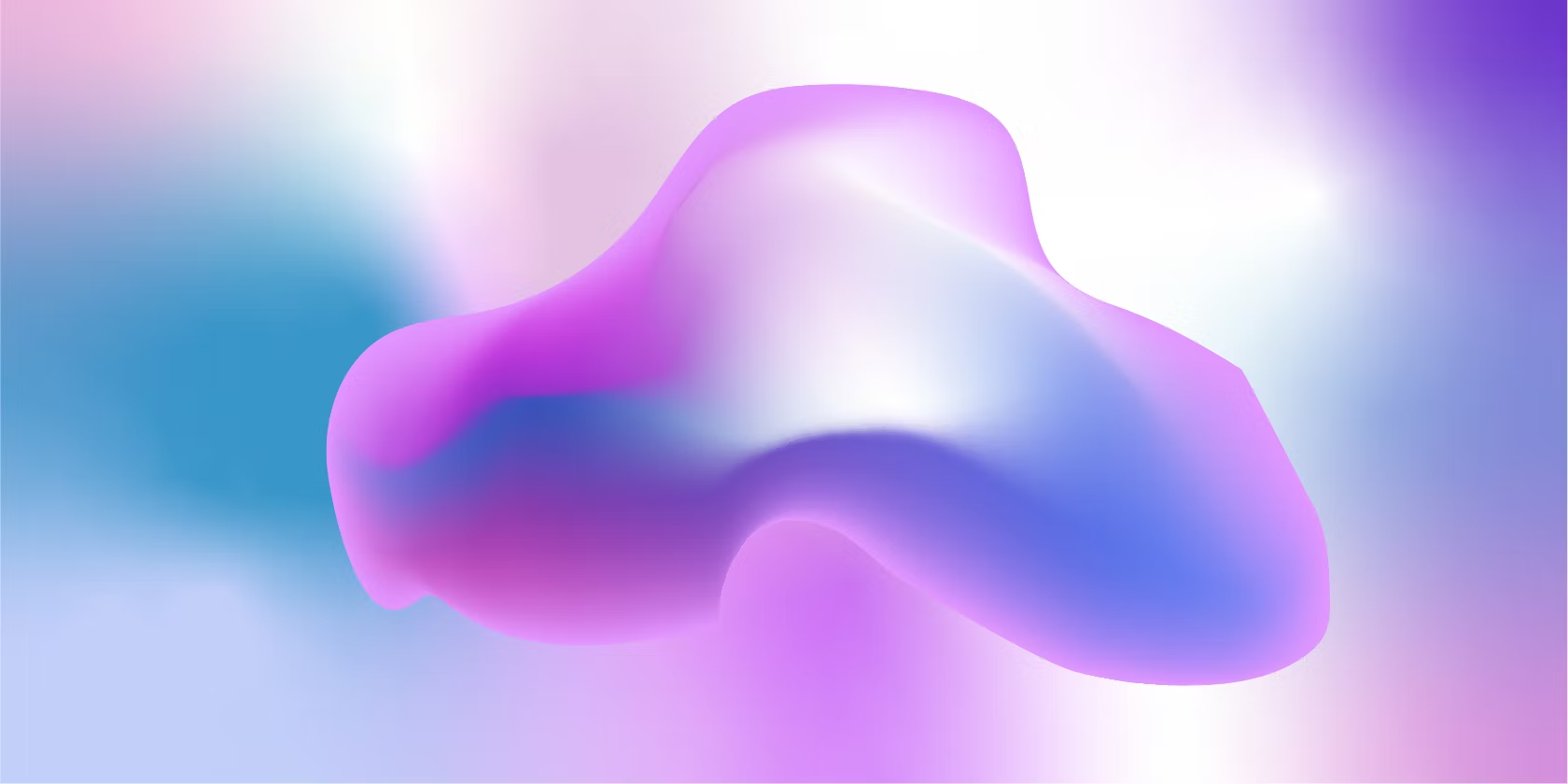
Gradient AI is a fascinating topic that blends the worlds of machine learning and data science. But what exactly is it? Gradient AI refers to the use of gradient-based algorithms to train artificial intelligence models. These algorithms help the AI learn by adjusting its parameters to minimize errors. Imagine teaching a robot to recognize cats by showing it thousands of pictures and tweaking its "brain" each time it makes a mistake. This process is what gradient-based learning does. It's like giving the AI a map and a compass to find the best route to the correct answer. Ready to dive into some cool facts about Gradient AI? Let's get started!
What is Gradient AI?
Gradient AI is a fascinating technology that combines artificial intelligence with gradient-based methods to solve complex problems. Here are some intriguing facts about Gradient AI that will help you understand its significance and applications.
-
Gradient AI uses gradient descent algorithms to optimize machine learning models. This method helps find the minimum of a function by iteratively moving towards the steepest descent.
-
It plays a crucial role in training neural networks. By adjusting weights and biases, Gradient AI ensures that the network learns from data efficiently.
-
Gradient AI is essential for backpropagation. This process calculates the gradient of the loss function with respect to each weight by the chain rule, which is vital for neural network training.
Applications of Gradient AI
Gradient AI has a wide range of applications across various fields. Let's explore some of the most notable ones.
-
In image recognition, Gradient AI helps in identifying objects within images by optimizing convolutional neural networks (CNNs).
-
It enhances natural language processing (NLP). By training models to understand and generate human language, Gradient AI improves tasks like translation, sentiment analysis, and chatbots.
-
Gradient AI is used in autonomous vehicles. It helps in training models to recognize and react to different driving scenarios, making self-driving cars safer and more reliable.
Benefits of Gradient AI
The advantages of using Gradient AI are numerous. Here are some key benefits that make it a popular choice among researchers and developers.
-
It improves model accuracy. By optimizing parameters, Gradient AI ensures that models make more accurate predictions.
-
Gradient AI speeds up training times. Efficient optimization algorithms reduce the time required to train complex models.
-
It reduces computational costs. By finding optimal solutions faster, Gradient AI minimizes the resources needed for training.
Challenges in Gradient AI
Despite its many benefits, Gradient AI also faces some challenges. Understanding these can help in developing better solutions.
-
Gradient vanishing and exploding problems. These issues occur when gradients become too small or too large, making it difficult for the model to learn.
-
Overfitting. Gradient AI models can sometimes learn the noise in the training data, leading to poor performance on new data.
-
Computational complexity. Training large models with Gradient AI can be resource-intensive and time-consuming.
Future of Gradient AI
The future of Gradient AI looks promising, with ongoing research and advancements. Here are some exciting developments to look forward to.
-
Improved optimization algorithms. Researchers are working on developing more efficient algorithms to overcome current challenges.
-
Integration with quantum computing. Combining Gradient AI with quantum computing could revolutionize optimization and problem-solving capabilities.
-
Enhanced interpretability. Efforts are being made to make Gradient AI models more transparent and understandable.
Real-World Examples of Gradient AI
Gradient AI is already making a significant impact in various industries. Here are some real-world examples of its applications.
-
Healthcare. Gradient AI helps in diagnosing diseases and predicting patient outcomes by analyzing medical data.
-
Finance. It is used in fraud detection, risk assessment, and algorithmic trading to make more informed decisions.
-
Gaming. Gradient AI enhances game development by creating more realistic and adaptive AI opponents.
Final Thoughts on Gradient AI
Gradient AI is transforming how we approach data analysis and machine learning. It simplifies complex tasks, making it easier for businesses to harness the power of artificial intelligence. With Gradient AI, companies can improve decision-making, optimize operations, and enhance customer experiences. This technology is not just for tech giants; small and medium-sized enterprises can also benefit from its capabilities. By integrating Gradient AI, organizations can stay competitive and innovative in an ever-changing market. The future of AI looks promising, and Gradient AI is at the forefront of this revolution. Embracing this technology can lead to significant advancements and opportunities. So, whether you're a business owner, a data scientist, or just curious about AI, Gradient AI offers tools and solutions that can make a real difference. Dive into the world of Gradient AI and see how it can transform your approach to data and machine learning.
Was this page helpful?
Our commitment to delivering trustworthy and engaging content is at the heart of what we do. Each fact on our site is contributed by real users like you, bringing a wealth of diverse insights and information. To ensure the highest standards of accuracy and reliability, our dedicated editors meticulously review each submission. This process guarantees that the facts we share are not only fascinating but also credible. Trust in our commitment to quality and authenticity as you explore and learn with us.