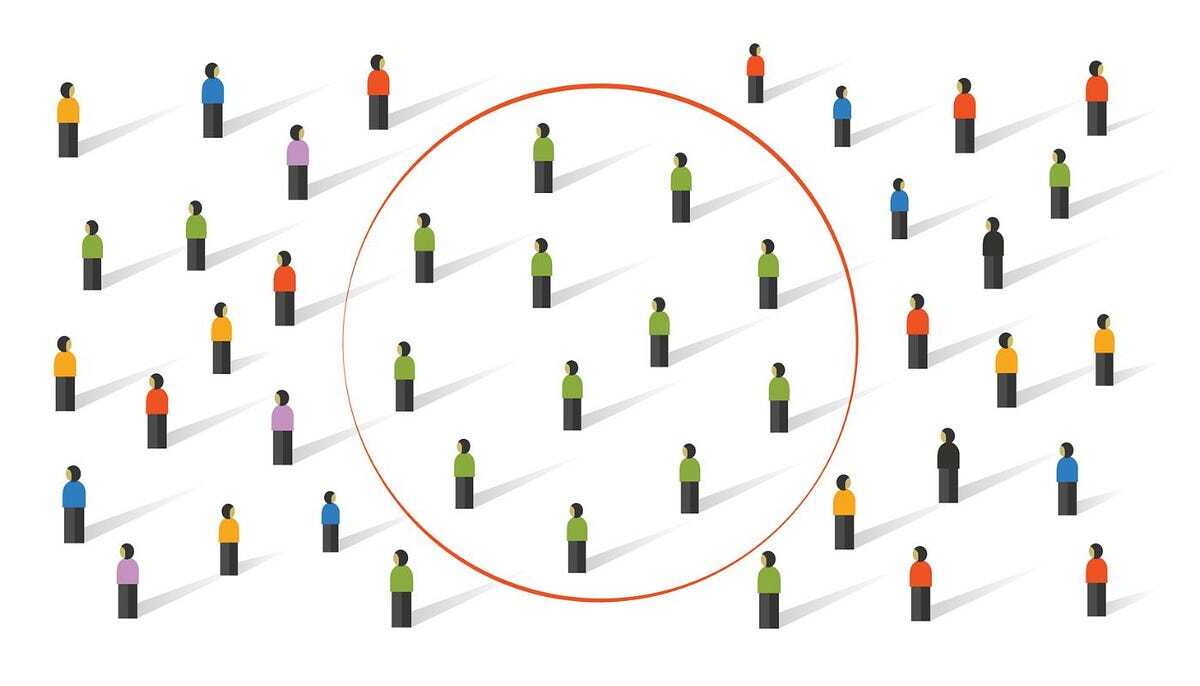
Algorithmic bias affects many aspects of our lives, often without us even realizing it. But what exactly is algorithmic bias? In simple terms, it's when computer systems make decisions that reflect human prejudices. These biases can creep into algorithms through the data used to train them or the way they are programmed. Imagine a hiring algorithm that favors certain resumes over others based on gender or ethnicity. This isn't just a tech issue; it impacts real people. Understanding algorithmic bias is crucial because it shapes everything from job opportunities to loan approvals. Ready to learn more? Let's dive into 18 eye-opening facts about this pressing issue.
What is Algorithmic Bias?
Algorithmic bias occurs when computer systems produce results that are systematically prejudiced due to erroneous assumptions in the machine learning process. These biases can have significant impacts on various aspects of society.
-
Bias in Data Collection: Algorithms learn from data. If the data collected is biased, the algorithm will likely reflect those biases. For example, if a hiring algorithm is trained on data from a company that has historically hired more men than women, it may favor male candidates.
-
Historical Bias: Historical biases are embedded in the data used to train algorithms. These biases can perpetuate existing inequalities. For instance, predictive policing algorithms may target minority communities more frequently if historical crime data is biased.
How Does Algorithmic Bias Affect Society?
Algorithmic bias can have far-reaching consequences, affecting everything from job opportunities to criminal justice outcomes. Understanding these impacts is crucial for developing fairer systems.
-
Employment Discrimination: Biased algorithms in hiring processes can lead to discrimination against certain groups. For example, an algorithm might favor resumes with traditionally male names over those with female names.
-
Healthcare Inequities: In healthcare, biased algorithms can result in unequal treatment. For instance, an algorithm designed to predict patient outcomes might underdiagnose certain conditions in minority populations due to lack of diverse training data.
-
Financial Inequality: Credit scoring algorithms can perpetuate financial inequality by denying loans to individuals from certain demographics based on biased data.
Examples of Algorithmic Bias in Action
Real-world examples highlight the pervasive nature of algorithmic bias and its potential to cause harm.
-
Facial Recognition: Facial recognition technology has been shown to have higher error rates for people with darker skin tones. This can lead to misidentification and wrongful accusations.
-
Predictive Policing: Predictive policing algorithms have been criticized for disproportionately targeting minority communities, leading to over-policing and increased tension between law enforcement and these communities.
-
Social Media Algorithms: Social media platforms use algorithms to curate content. These algorithms can create echo chambers, reinforcing existing biases and spreading misinformation.
Efforts to Mitigate Algorithmic Bias
Addressing algorithmic bias requires concerted efforts from researchers, policymakers, and technology companies. Various strategies are being employed to tackle this issue.
-
Diverse Data Sets: One approach to reducing bias is to use more diverse data sets when training algorithms. This can help ensure that the algorithm performs well across different demographic groups.
-
Bias Audits: Regular audits of algorithms can help identify and mitigate biases. These audits involve testing the algorithm on various data sets to check for biased outcomes.
-
Transparency: Increasing transparency in how algorithms are developed and used can help identify biases. Open-source algorithms allow for greater scrutiny and collaboration in addressing bias.
The Role of Regulation in Combating Algorithmic Bias
Regulation can play a crucial role in ensuring that algorithms are fair and unbiased. Governments and regulatory bodies are beginning to take action in this area.
-
Anti-Discrimination Laws: Existing anti-discrimination laws can be applied to algorithms to ensure they do not perpetuate bias. For example, the Equal Employment Opportunity Commission (EEOC) in the U.S. can investigate biased hiring algorithms.
-
Algorithmic Accountability: Some jurisdictions are introducing laws that require companies to be accountable for the algorithms they use. This includes disclosing how algorithms make decisions and ensuring they do not discriminate.
-
Ethical Guidelines: Ethical guidelines for AI development can help prevent bias. Organizations like the IEEE and the European Commission have developed guidelines to promote fairness and transparency in AI.
The Future of Algorithmic Bias
As technology evolves, so too will the challenges and solutions related to algorithmic bias. Ongoing research and innovation are essential for creating fairer algorithms.
-
AI Ethics Research: Continued research in AI ethics is crucial for understanding and mitigating bias. This includes studying how biases arise and developing methods to prevent them.
-
Human Oversight: Human oversight of algorithms can help catch biases that automated systems might miss. This involves having humans review algorithmic decisions and intervene when necessary.
-
Collaborative Efforts: Collaboration between tech companies, researchers, and policymakers is essential for addressing algorithmic bias. By working together, these stakeholders can develop more effective solutions.
-
Public Awareness: Increasing public awareness of algorithmic bias can drive demand for fairer algorithms. Educating people about the issue can lead to greater scrutiny and pressure on companies to address biases.
The Bigger Picture
Algorithmic bias is a real issue that affects many aspects of our lives. From hiring practices to loan approvals, these biases can have serious consequences. Understanding how algorithms work and recognizing their limitations is crucial. By being aware of these biases, we can push for more fairness and transparency in technology. It's not just about fixing the code; it's about changing the mindset behind it. Everyone has a role to play, whether you're a developer, a policymaker, or just someone who uses technology daily. Let's strive for a future where technology serves everyone equally. Keep questioning, keep learning, and don't settle for less. The more we know, the better we can demand improvements. So next time you interact with an algorithm, remember there's always room for improvement.
Was this page helpful?
Our commitment to delivering trustworthy and engaging content is at the heart of what we do. Each fact on our site is contributed by real users like you, bringing a wealth of diverse insights and information. To ensure the highest standards of accuracy and reliability, our dedicated editors meticulously review each submission. This process guarantees that the facts we share are not only fascinating but also credible. Trust in our commitment to quality and authenticity as you explore and learn with us.