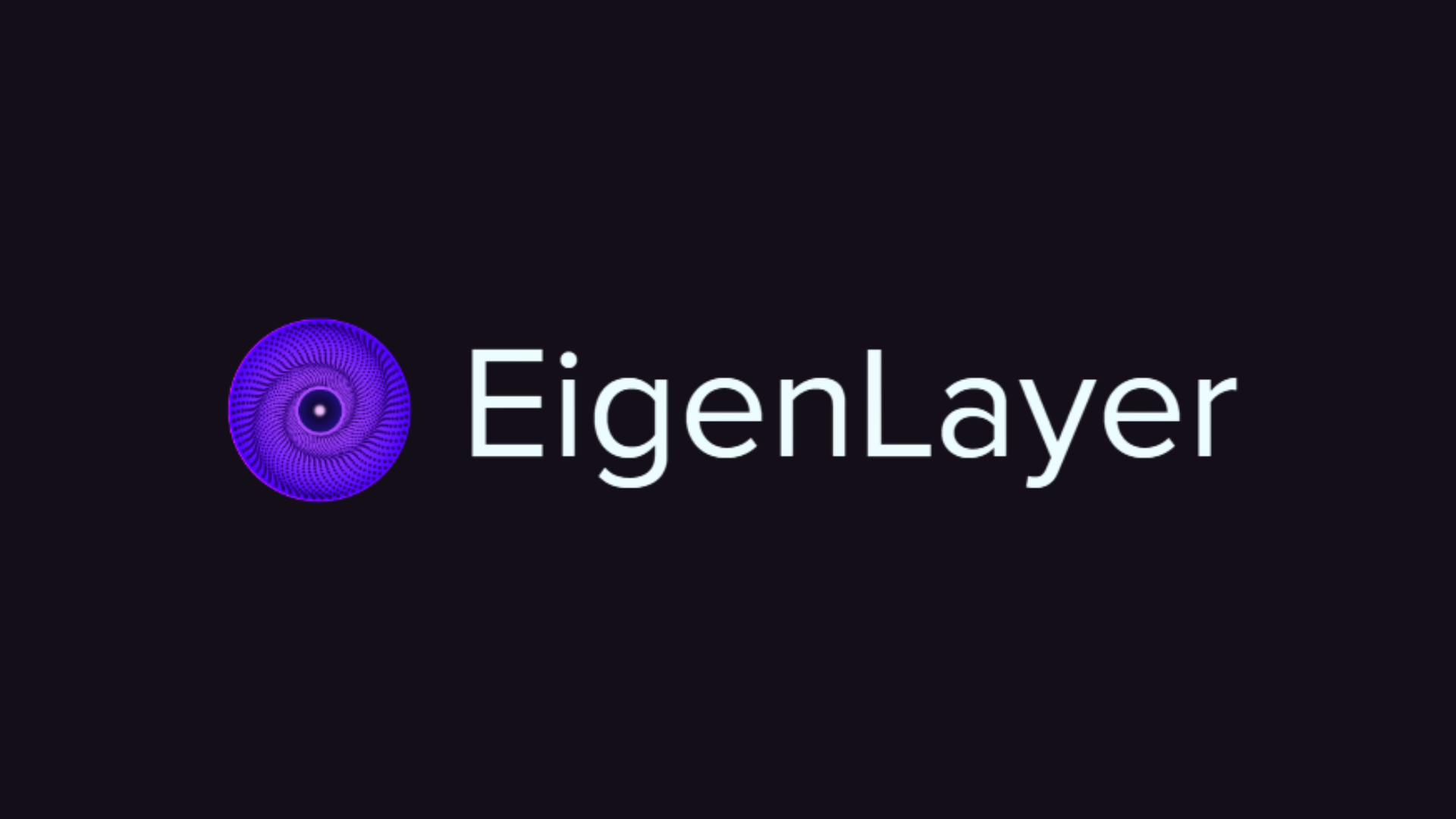
Eigenlayers are a fundamental concept in the realm of machine learning and artificial intelligence. These layers play a pivotal role in the process of extracting meaningful features from raw data, thereby enabling the development of sophisticated models capable of making accurate predictions and classifications. Understanding eigenlayers is crucial for anyone delving into the intricacies of neural networks and deep learning.
In this article, we will delve into the world of eigenlayers, unraveling their significance and shedding light on their applications across various domains. From their mathematical underpinnings to their practical implications, we will explore nine compelling facts about eigenlayers, providing you with a comprehensive understanding of this essential component of modern machine learning. So, let's embark on this enlightening journey to uncover the essence of eigenlayers and their profound impact on the ever-evolving landscape of technology and innovation.
Key Takeaways:
- Eigenlayer is like a superhero for machine learning, helping to extract important features from data and make neural networks smarter and more efficient.
- By using Eigenlayer, machine learning models become easier to understand and more powerful, driving the development of advanced AI systems and pushing the boundaries of deep learning research.
Eigenlayer is a fundamental concept in machine learning.
Eigenlayer is a fundamental concept in machine learning, particularly in the field of neural networks. It plays a crucial role in the process of dimensionality reduction and feature extraction.
Eigenlayer is utilized in various deep learning applications.
Eigenlayer is widely utilized in various deep learning applications, including image recognition, natural language processing, and speech recognition.
Eigenlayer enables the extraction of essential features from data.
Eigenlayer enables the extraction of essential features from data, allowing for more efficient and effective processing in machine learning models.
Eigenlayer is based on the mathematical concept of eigenvectors and eigenvalues.
Eigenlayer is based on the mathematical concept of eigenvectors and eigenvalues, which are essential in linear algebra and have significant applications in machine learning and data analysis.
Eigenlayer contributes to the optimization of neural network performance.
Eigenlayer contributes to the optimization of neural network performance by reducing the dimensionality of data and enhancing the model's ability to identify and learn important patterns.
Eigenlayer aids in reducing computational complexity.
Eigenlayer aids in reducing computational complexity by transforming the input data into a more manageable form, thereby improving the efficiency of machine learning algorithms.
Eigenlayer enhances the interpretability of machine learning models.
Eigenlayer enhances the interpretability of machine learning models by extracting meaningful features from complex data, leading to improved understanding and insights into the underlying patterns.
Eigenlayer is a key component in the development of advanced AI systems.
Eigenlayer is a key component in the development of advanced AI systems, contributing to the evolution of intelligent technologies and their applications across various industries.
Eigenlayer plays a pivotal role in the advancement of deep learning research.
Eigenlayer plays a pivotal role in the advancement of deep learning research, driving innovation and progress in the field of artificial intelligence and machine learning.
Eigenlayer, a fundamental concept in machine learning, is essential for dimensionality reduction and feature extraction in neural networks. It is widely utilized in various deep learning applications, including image recognition, natural language processing, and speech recognition. Based on the mathematical concept of eigenvectors and eigenvalues, Eigenlayer enables the extraction of essential features from data, contributing to the optimization of neural network performance and the reduction of computational complexity. Moreover, Eigenlayer enhances the interpretability of machine learning models and is a key component in the development of advanced AI systems. Its pivotal role in the advancement of deep learning research underscores its significance in driving innovation and progress in the field of artificial intelligence and machine learning.
Conclusion
In conclusion, Eigenlayer is a fascinating concept that plays a crucial role in various fields, including machine learning, signal processing, and neural networks. Understanding the fundamental aspects of Eigenlayers, such as their ability to capture essential features and their impact on data representation, is essential for professionals and enthusiasts in these domains. As technology continues to advance, Eigenlayers are likely to become even more integral to the development of innovative solutions and applications. Embracing the potential of Eigenlayers can lead to groundbreaking advancements in artificial intelligence and data analysis, opening up new possibilities for solving complex problems and enhancing computational efficiency.
FAQs
What is the significance of Eigenlayers in machine learning?Eigenlayers are significant in machine learning as they enable the extraction of essential features from data, contributing to more effective and efficient learning processes. By leveraging Eigenlayers, machine learning models can better comprehend complex patterns and make accurate predictions, ultimately enhancing their performance across various tasks.
How are Eigenlayers utilized in signal processing?Eigenlayers are utilized in signal processing to analyze and extract crucial information from signals, such as audio, images, and sensor data. By applying Eigenlayer techniques, signal processing systems can effectively reduce noise, identify patterns, and enhance the overall quality of the processed signals, leading to improved outcomes in diverse applications, from audio enhancement to image recognition.
Was this page helpful?
Our commitment to delivering trustworthy and engaging content is at the heart of what we do. Each fact on our site is contributed by real users like you, bringing a wealth of diverse insights and information. To ensure the highest standards of accuracy and reliability, our dedicated editors meticulously review each submission. This process guarantees that the facts we share are not only fascinating but also credible. Trust in our commitment to quality and authenticity as you explore and learn with us.