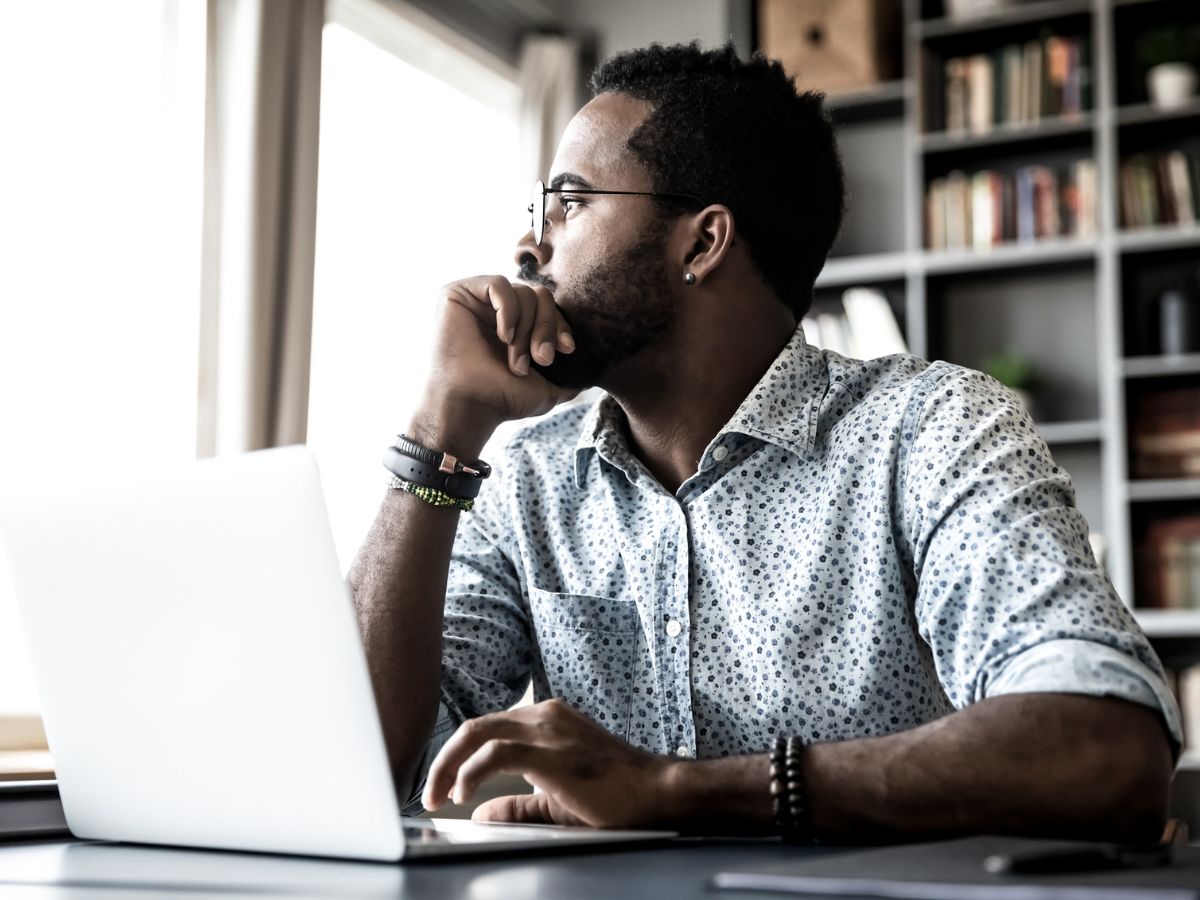
Deep learning is transforming how machines interpret the world around us. From voice recognition in smartphones to self-driving cars, this technology is behind some of today's most exciting innovations. But what exactly is deep learning, and why is it so groundbreaking? At its core, deep learning is a subset of machine learning, utilizing neural networks with many layers to process data in complex ways. This introduction will guide you through 20 fascinating facts about deep learning, shedding light on its capabilities, how it's changing industries, and its potential for the future. Whether you're a tech enthusiast or simply curious about the advancements in artificial intelligence, these insights will provide a comprehensive overview of deep learning's role in modern technology.
What is Deep Learning?
Deep learning, a subset of machine learning, mimics the workings of the human brain in processing data for use in detecting objects, recognizing speech, translating languages, and making decisions. Unlike traditional machine learning algorithms, which linearly analyze data, deep learning utilizes a hierarchical level of artificial neural networks to carry out the process of machine learning. The neural networks are designed to imitate the human brain's operations, allowing these machines to think and learn more like humans.
-
Deep learning models can learn directly from data. They can automatically discover the representations needed for feature detection or classification, without human intervention.
-
The term "deep" in deep learning refers to the number of layers through which the data is transformed. More layers allow for more complex features to be extracted and learned.
How Does Deep Learning Work?
At its core, deep learning involves feeding a computer system a lot of data, which it then uses to make decisions about other data. This data is fed through neural networks, which are complex algorithms modeled after the human brain. As data passes through each layer of the network, the system learns to understand features and patterns more deeply.
-
Neural networks consist of neurons with learnable weights and biases. Each neuron receives several inputs, takes a weighted sum over them, passes it through an activation function, and responds with an output.
-
Deep learning networks require a large amount of data to learn effectively. However, once trained, they can outperform humans at tasks like image recognition and voice recognition.
Applications of Deep Learning
Deep learning has been successfully applied in various fields, including computer vision, speech recognition, natural language processing, and even in areas like healthcare and autonomous vehicles.
-
In healthcare, deep learning algorithms can analyze medical images to help diagnose diseases with high accuracy, sometimes even before symptoms have developed.
-
Autonomous vehicles use deep learning to detect objects around them, making decisions about when to speed up, slow down, or steer to avoid collisions.
Challenges in Deep Learning
While deep learning offers significant advantages, it also comes with its set of challenges.
-
Deep learning models require vast amounts of labeled data to train. This can be a significant barrier for some applications where data is scarce or hard to come by.
-
These models are often described as "black boxes" because their decision-making process is not always transparent, making it difficult to understand how they arrive at certain conclusions.
Future of Deep Learning
The future of deep learning is incredibly promising, with ongoing research pushing the boundaries of what's possible.
-
Advancements in deep learning could lead to more sophisticated AI that can perform tasks currently beyond our capabilities, such as more complex decision-making or understanding human emotions better.
-
Researchers are also working on making deep learning models more efficient and less data-hungry, which could enable their deployment in a wider range of applications.
Deep Learning vs. Machine Learning
It's essential to understand the distinction between deep learning and the broader field of machine learning.
-
All deep learning is machine learning, but not all machine learning is deep learning. Deep learning is just one approach to machine learning that has proven particularly powerful for certain types of problems.
-
Machine learning algorithms are typically designed to learn to do tasks based on rules and logic programmed by humans. In contrast, deep learning algorithms learn from vast amounts of data, identifying patterns and making decisions with minimal human intervention.
Key Components of Deep Learning
Deep learning architectures are complex and varied, but there are key components that are foundational to most deep learning systems.
-
Activation functions are critical in neural networks, helping models learn complex patterns. They determine whether a neuron should be activated or not, allowing the model to make more sophisticated decisions.
-
Convolutional Neural Networks (CNNs) are a type of deep neural network especially effective for image recognition and similar tasks. They are designed to automatically and adaptively learn spatial hierarchies of features from images.
-
Recurrent Neural Networks (RNNs) are another type of neural network useful for processing sequences, such as sentences, making them ideal for tasks in natural language processing.
The Impact of Deep Learning
The impact of deep learning technology is vast and still unfolding.
-
Deep learning has significantly improved the accuracy of systems in fields like speech recognition and computer vision, surpassing human-level performance in some tasks.
-
It has democratized the creation of sophisticated AI tools, making powerful technology accessible to more people and organizations.
-
The technology has also sparked ethical debates, particularly around privacy, surveillance, and the potential for bias in decision-making processes.
Deep Learning Tools and Libraries
Several tools and libraries have been developed to facilitate deep learning research and application.
-
TensorFlow and PyTorch are among the most popular frameworks for deep learning. They offer comprehensive libraries for designing, training, and deploying deep learning models.
-
Keras is a high-level neural networks API, written in Python and capable of running on top of TensorFlow, CNTK, or Theano. It allows for easy and fast prototyping of deep learning models.
A Final Look at Deep Learning's Wonders
Deep learning has truly transformed how machines interpret the world. From self-driving cars to virtual assistants, its impact is undeniable. This technology, rooted in neural networks, mimics human brain functions, enabling machines to learn from vast amounts of data. Its applications are vast, touching every industry, including healthcare, finance, and entertainment. As we've seen, deep learning's capabilities are not just about automating tasks but also about enhancing our understanding of complex patterns and making more informed decisions. The journey of deep learning is far from over. With ongoing research and development, its potential is boundless. It's an exciting time to witness how deep learning will continue to evolve and shape our future. Let's keep an eye on this fascinating field as it unfolds new possibilities and challenges.
Was this page helpful?
Our commitment to delivering trustworthy and engaging content is at the heart of what we do. Each fact on our site is contributed by real users like you, bringing a wealth of diverse insights and information. To ensure the highest standards of accuracy and reliability, our dedicated editors meticulously review each submission. This process guarantees that the facts we share are not only fascinating but also credible. Trust in our commitment to quality and authenticity as you explore and learn with us.