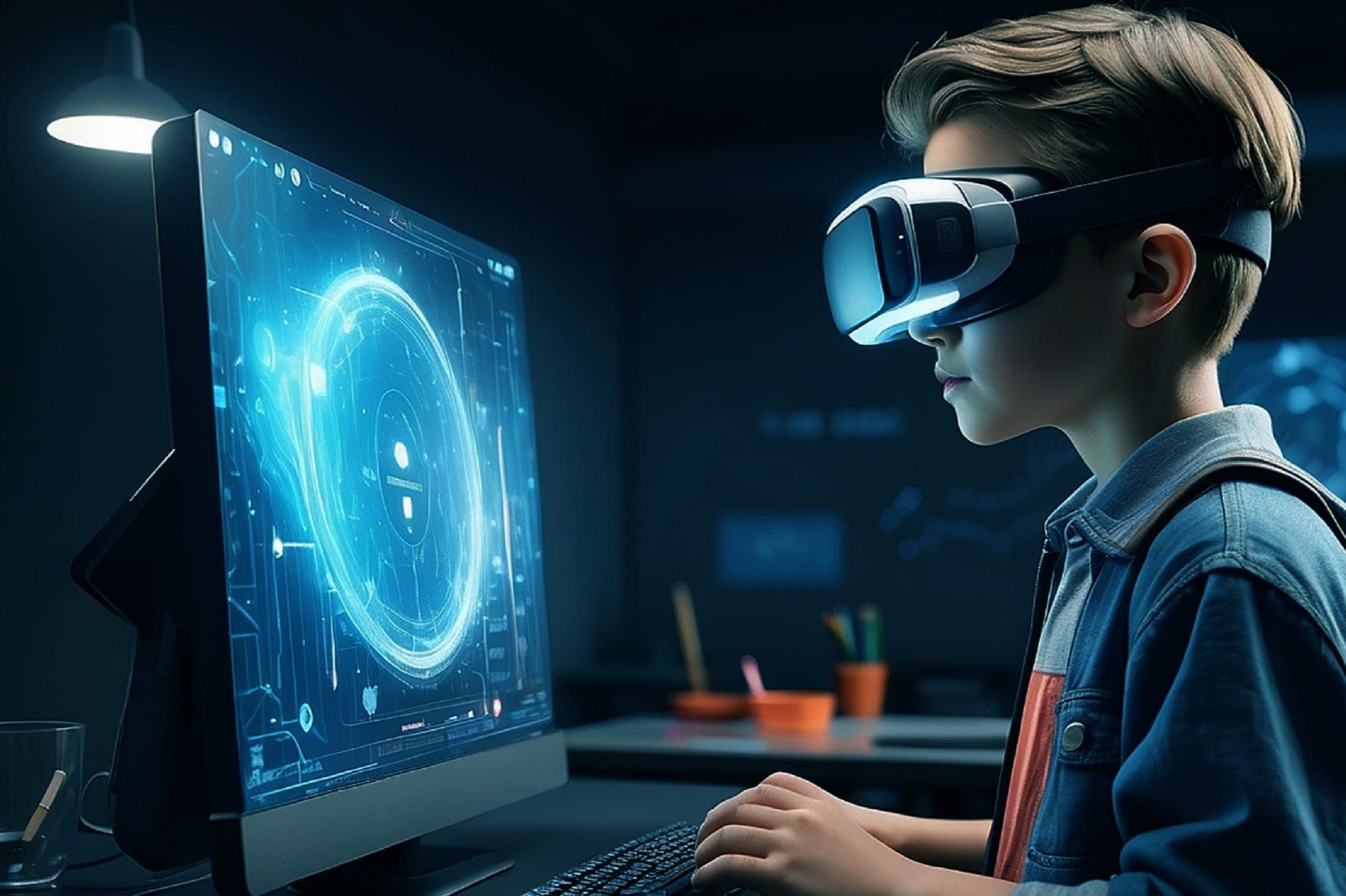
Zero-shot learning is a fascinating concept in artificial intelligence that allows models to recognize objects or perform tasks without having seen any examples during training. Imagine teaching a computer to identify a zebra without ever showing it a picture of one! This approach leverages existing knowledge to make educated guesses about new, unseen data. Zero-shot learning is transforming fields like natural language processing, image recognition, and even gaming. It opens up possibilities for more adaptable and intelligent systems that can handle a wider range of tasks with minimal human intervention. Ready to dive into the world of zero-shot learning? Let's explore 19 intriguing facts about this cutting-edge technology!
What is Zero-Shot Learning?
Zero-shot learning (ZSL) is a fascinating concept in machine learning. It allows models to recognize and classify data they haven't encountered before. This technique is transforming how artificial intelligence (AI) systems operate.
-
Zero-shot learning enables AI to identify objects or concepts without prior training on those specific items. Imagine a model that can recognize a new animal species without having seen any images of it before.
-
ZSL relies heavily on semantic information. This means it uses descriptions, attributes, or relationships to understand new categories. For instance, knowing that a zebra is a horse-like animal with black and white stripes helps the model identify it.
How Zero-Shot Learning Works
Understanding the mechanics behind ZSL can shed light on its potential and limitations. Here are some key points about its working principles.
-
ZSL uses a shared feature space. Both seen and unseen classes are mapped into this space, allowing the model to generalize from known to unknown categories.
-
Attribute-based learning is crucial for ZSL. Models learn attributes like color, shape, or size, which helps in identifying new objects. For example, knowing that a flamingo is pink and has long legs aids in its recognition.
-
Natural language processing (NLP) plays a significant role. Descriptions and textual data help the model understand new categories. Think of it as reading a description of an animal and then being able to recognize it.
Applications of Zero-Shot Learning
ZSL isn't just a theoretical concept; it has practical applications across various fields. Let's explore some of these applications.
-
Image and video recognition benefit greatly from ZSL. It allows systems to identify new objects in images or videos without needing extensive training data.
-
Healthcare uses ZSL for diagnosing rare diseases. Doctors can input symptoms or descriptions, and the AI can suggest possible conditions, even if it hasn't encountered them before.
-
ZSL enhances natural language understanding. Virtual assistants and chatbots can understand and respond to queries about topics they haven't been explicitly trained on.
Challenges in Zero-Shot Learning
Despite its potential, ZSL faces several challenges that researchers are working to overcome. Here are some of the main hurdles.
-
Semantic gap is a significant issue. The difference between the feature space of seen and unseen classes can lead to misclassification.
-
Data quality and quantity matter. Poorly described attributes or insufficient data can hamper the model's performance.
-
Scalability is a concern. As the number of unseen classes increases, maintaining accuracy becomes more challenging.
Future of Zero-Shot Learning
The future of ZSL looks promising, with ongoing research and advancements. Here are some trends and predictions for its development.
-
Integration with other AI techniques is on the rise. Combining ZSL with transfer learning or few-shot learning can enhance its capabilities.
-
Improved semantic representations are being developed. Better ways to describe and understand attributes will help bridge the semantic gap.
-
Real-world applications are expanding. From autonomous vehicles to personalized recommendations, ZSL is finding new uses.
Real-World Examples of Zero-Shot Learning
Seeing ZSL in action can help illustrate its impact and potential. Here are some real-world examples where ZSL is making a difference.
-
Google's image search uses ZSL. It can identify and categorize images even if they haven't been explicitly labeled.
-
Microsoft's AI for Earth initiative employs ZSL. It helps in identifying and protecting endangered species by recognizing them from limited data.
-
E-commerce platforms use ZSL for product recommendations. They can suggest items based on descriptions and attributes, even if those items haven't been previously sold.
Research and Development in Zero-Shot Learning
Ongoing research is crucial for the advancement of ZSL. Here are some areas where researchers are focusing their efforts.
-
Improving attribute learning is a key focus. Researchers are developing better ways to teach models about attributes and their relationships.
-
Cross-modal learning is gaining attention. This involves using data from different sources, like images and text, to improve ZSL performance.
The Future of Zero-Shot Learning
Zero-shot learning (ZSL) is changing how machines understand and process information. By enabling models to recognize and classify data they haven't seen before, ZSL opens up new possibilities in artificial intelligence. This approach reduces the need for extensive labeled datasets, saving time and resources. Industries like healthcare, finance, and entertainment are already benefiting from these advancements.
As ZSL continues to evolve, its applications will expand, making AI more versatile and efficient. Researchers are constantly improving algorithms to enhance accuracy and reliability. This progress means better performance in real-world scenarios, from diagnosing diseases to predicting market trends.
Understanding ZSL's potential helps us appreciate the strides being made in AI. Staying informed about these developments ensures we're ready to harness the power of this technology in various fields. Zero-shot learning isn't just a buzzword; it's a glimpse into the future of intelligent systems.
Was this page helpful?
Our commitment to delivering trustworthy and engaging content is at the heart of what we do. Each fact on our site is contributed by real users like you, bringing a wealth of diverse insights and information. To ensure the highest standards of accuracy and reliability, our dedicated editors meticulously review each submission. This process guarantees that the facts we share are not only fascinating but also credible. Trust in our commitment to quality and authenticity as you explore and learn with us.