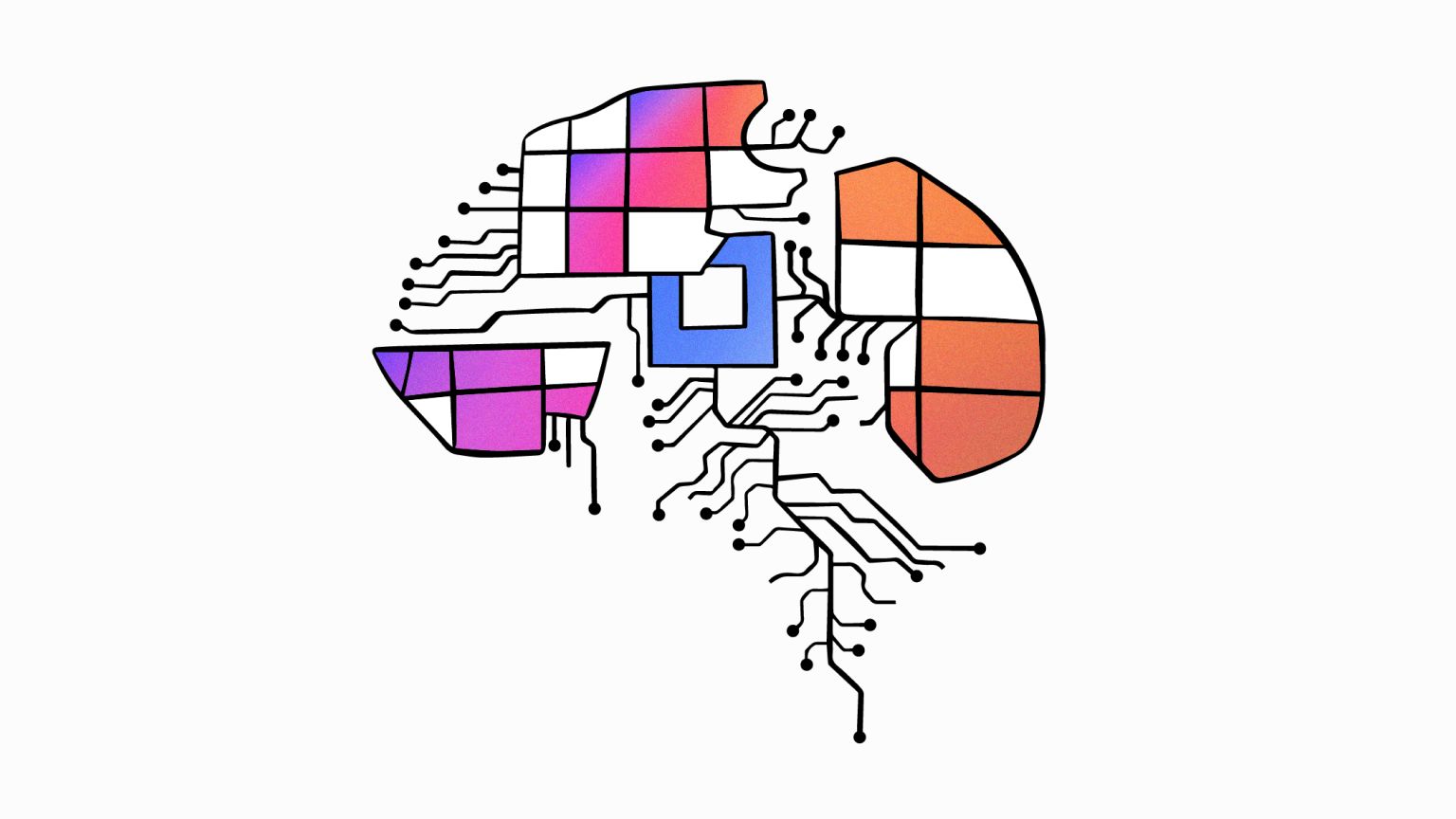
Few-shot learning is a fascinating area of machine learning that allows models to learn from just a few examples. Imagine teaching a child to recognize a new animal by showing only a couple of pictures. Few-shot learning aims to achieve similar feats with artificial intelligence. This approach is particularly useful in situations where collecting large amounts of data is impractical or impossible. It leverages prior knowledge to make educated guesses, reducing the need for extensive datasets. In this blog post, we’ll explore 17 intriguing facts about few-shot learning, shedding light on its potential, challenges, and real-world applications. Whether you're a tech enthusiast or just curious, these insights will help you understand why few-shot learning is a game-changer in AI.
What is Few-Shot Learning?
Few-shot learning is a type of machine learning where models are trained to recognize patterns with very few examples. This approach mimics human learning, where we can often generalize from limited data.
-
Few-shot learning can drastically reduce the amount of data needed for training, making it ideal for situations where data is scarce.
-
It uses techniques like meta-learning to help models learn how to learn, enabling them to adapt quickly to new tasks with minimal data.
How Does Few-Shot Learning Work?
Understanding the mechanics behind few-shot learning can help grasp its potential and limitations. Here are some key aspects.
-
Few-shot learning often relies on transfer learning, where a model pre-trained on a large dataset is fine-tuned on a smaller, task-specific dataset.
-
Siamese networks are commonly used in few-shot learning. These networks compare pairs of inputs to determine if they belong to the same category.
Applications of Few-Shot Learning
Few-shot learning has a wide range of applications, from healthcare to natural language processing. Here are some examples.
-
In medical imaging, few-shot learning can help identify rare diseases by training models on a limited number of medical images.
-
Voice recognition systems benefit from few-shot learning by quickly adapting to new users with minimal voice samples.
Challenges in Few-Shot Learning
Despite its advantages, few-shot learning faces several challenges that researchers are working to overcome.
-
One major challenge is overfitting, where the model performs well on the training data but poorly on new, unseen data.
-
Data quality is crucial in few-shot learning. Poor-quality data can lead to inaccurate models, making it essential to have clean, well-labeled data.
Few-Shot Learning vs. Traditional Machine Learning
Comparing few-shot learning with traditional machine learning methods highlights its unique advantages and limitations.
-
Traditional machine learning requires large datasets for training, whereas few-shot learning can work with as few as one or two examples.
-
Few-shot learning models are generally more flexible and can adapt to new tasks faster than traditional models.
Future of Few-Shot Learning
The future of few-shot learning looks promising, with ongoing research and development aimed at overcoming current limitations.
-
Automated machine learning (AutoML) is expected to integrate few-shot learning techniques, making it easier for non-experts to build effective models.
-
Advances in neural architecture search (NAS) could lead to the discovery of new model architectures optimized for few-shot learning.
Real-World Examples of Few-Shot Learning
Few-shot learning is already making an impact in various industries. Here are some real-world examples.
-
Google's AI uses few-shot learning to improve its search algorithms, providing more accurate results with less data.
-
Facebook employs few-shot learning to enhance its facial recognition technology, making it more efficient and accurate.
Few-Shot Learning in Natural Language Processing
Natural language processing (NLP) is another field where few-shot learning is making significant strides.
-
Few-shot learning helps in text classification, enabling models to categorize text with minimal labeled examples.
-
Chatbots and virtual assistants use few-shot learning to understand and respond to new queries without extensive retraining.
Few-Shot Learning in Computer Vision
Computer vision applications are also benefiting from few-shot learning techniques.
- Object detection systems can identify new objects with just a few labeled images, making them more versatile and efficient.
The Power of Few-Shot Learning
Few-shot learning is a game-changer in artificial intelligence. It allows models to learn from just a handful of examples, making it incredibly efficient. This technique is especially useful in situations where data is scarce or expensive to obtain. By leveraging few-shot learning, AI can quickly adapt to new tasks, saving time and resources.
Moreover, it opens up possibilities for personalized applications, where models can be fine-tuned with minimal data. This adaptability makes few-shot learning a valuable tool in various fields, from healthcare to finance. As AI continues to evolve, few-shot learning will likely play a crucial role in its development.
Understanding the basics and potential of few-shot learning can help you stay ahead in the ever-changing landscape of technology. So, keep an eye on this exciting area of AI research. It’s bound to bring more innovations in the near future.
Was this page helpful?
Our commitment to delivering trustworthy and engaging content is at the heart of what we do. Each fact on our site is contributed by real users like you, bringing a wealth of diverse insights and information. To ensure the highest standards of accuracy and reliability, our dedicated editors meticulously review each submission. This process guarantees that the facts we share are not only fascinating but also credible. Trust in our commitment to quality and authenticity as you explore and learn with us.