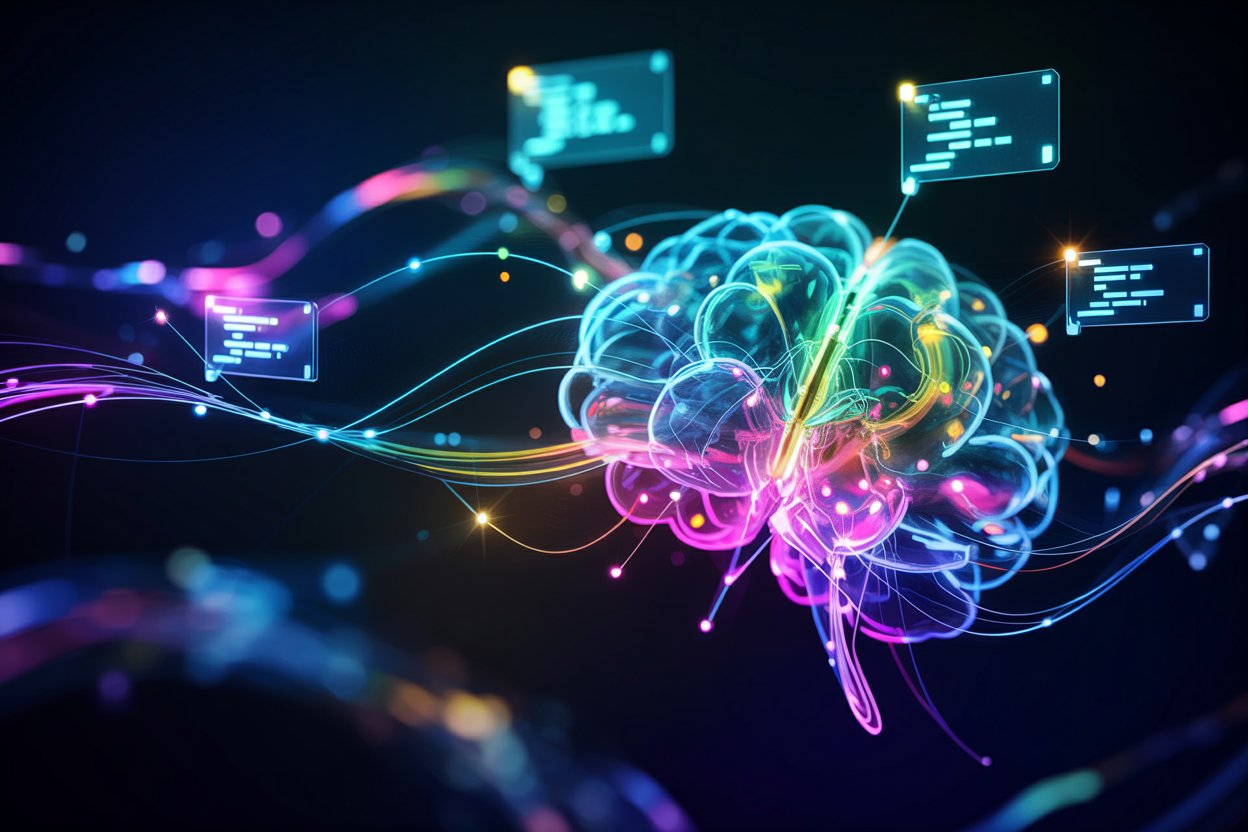
InfoGAN stands for Information Maximizing Generative Adversarial Network. It's a type of neural network designed to generate realistic data, like images or text, while also learning to represent the underlying structure of that data. InfoGAN achieves this by maximizing mutual information between a subset of the latent variables and the observations. This means it can produce more interpretable and controllable outputs compared to traditional GANs. InfoGAN has found applications in various fields, including image synthesis, data augmentation, and even unsupervised learning. Understanding InfoGAN can open doors to creating more sophisticated AI models that can learn and generate data in a more meaningful way.
What is InfoGAN?
InfoGAN is a fascinating concept in the world of artificial intelligence and machine learning. It stands for Information Maximizing Generative Adversarial Network. This innovative approach aims to improve the quality and interpretability of generated data. Let's dive into some intriguing facts about InfoGAN.
- 01
InfoGAN is a type of GAN: Generative Adversarial Networks (GANs) are a class of machine learning frameworks designed by Ian Goodfellow and his colleagues in 2014. InfoGAN is a variant that focuses on maximizing mutual information.
- 02
Introduced in 2016: InfoGAN was introduced by Xi Chen, Yan Duan, and their team in a paper published in 2016. Their work aimed to enhance the interpretability of the latent variables in GANs.
- 03
Mutual Information Maximization: InfoGAN maximizes mutual information between a subset of the latent variables and the observations. This helps in learning interpretable and meaningful representations.
- 04
Unsupervised Learning: InfoGAN operates in an unsupervised learning setting, meaning it doesn't require labeled data to train. This makes it highly versatile for various applications.
- 05
Latent Codes: InfoGAN introduces latent codes that can control different aspects of the generated data. These codes help in understanding and manipulating the features of the generated samples.
How InfoGAN Works
Understanding the working mechanism of InfoGAN can be quite enlightening. It involves a few key steps and components that make it unique.
- 06
Generator and Discriminator: Like traditional GANs, InfoGAN consists of a generator and a discriminator. The generator creates fake data, while the discriminator tries to distinguish between real and fake data.
- 07
Latent Variables: InfoGAN uses latent variables to encode information. These variables are divided into two parts: noise variables and code variables.
- 08
Mutual Information Loss: A mutual information loss term is added to the GAN's objective function. This term encourages the generator to produce data that is informative about the latent codes.
- 09
Regularization: InfoGAN uses regularization techniques to ensure that the latent codes remain informative and interpretable.
- 10
Training Process: The training process involves alternating between updating the generator and the discriminator, similar to traditional GANs. However, InfoGAN also updates the mutual information term.
Applications of InfoGAN
InfoGAN has a wide range of applications, thanks to its ability to generate interpretable and high-quality data. Here are some areas where InfoGAN shines.
- 11
Image Generation: InfoGAN can generate high-quality images with controllable features. This makes it useful for tasks like image synthesis and editing.
- 12
Data Augmentation: InfoGAN can be used to augment datasets by generating new samples. This is particularly useful in scenarios where labeled data is scarce.
- 13
Style Transfer: InfoGAN can perform style transfer by manipulating the latent codes. This allows for changing the style of an image while preserving its content.
- 14
Anomaly Detection: InfoGAN can help in detecting anomalies by learning the distribution of normal data and identifying deviations from it.
- 15
Text Generation: InfoGAN can be applied to text generation tasks, where it can generate coherent and contextually relevant text.
Advantages of InfoGAN
InfoGAN offers several advantages over traditional GANs and other generative models. These benefits make it a popular choice among researchers and practitioners.
- 16
Interpretability: One of the main advantages of InfoGAN is its interpretability. The latent codes provide meaningful and controllable features.
- 17
High-Quality Data: InfoGAN can generate high-quality data that is often indistinguishable from real data.
- 18
Versatility: InfoGAN is versatile and can be applied to various types of data, including images, text, and audio.
- 19
Unsupervised Learning: InfoGAN's ability to learn without labeled data makes it highly valuable in many real-world scenarios.
- 20
Improved Training Stability: InfoGAN often exhibits improved training stability compared to traditional GANs, thanks to the mutual information term.
Challenges and Limitations
Despite its many advantages, InfoGAN also faces some challenges and limitations. Understanding these can help in better utilizing the model.
- 21
Complexity: InfoGAN is more complex to implement and train compared to traditional GANs. The additional mutual information term adds to the complexity.
- 22
Computational Resources: Training InfoGAN can be computationally intensive, requiring significant resources.
- 23
Hyperparameter Tuning: InfoGAN requires careful tuning of hyperparameters to achieve optimal performance.
- 24
Mode Collapse: Like other GANs, InfoGAN can suffer from mode collapse, where the generator produces limited diversity in the generated data.
- 25
Scalability: Scaling InfoGAN to very large datasets or high-resolution data can be challenging.
Future Directions
The field of generative models is rapidly evolving, and InfoGAN is no exception. Here are some potential future directions for InfoGAN research and development.
- 26
Improved Architectures: Researchers are continually working on improving the architectures of InfoGAN to enhance performance and stability.
- 27
Hybrid Models: Combining InfoGAN with other models, such as Variational Autoencoders (VAEs), could lead to more powerful generative models.
- 28
Real-World Applications: Exploring new real-world applications of InfoGAN, such as in healthcare and finance, could unlock new possibilities.
- 29
Better Regularization Techniques: Developing better regularization techniques to address issues like mode collapse and overfitting.
- 30
Scalability Solutions: Finding solutions to improve the scalability of InfoGAN for large-scale and high-resolution data.
- 31
Interpretable AI: InfoGAN's focus on interpretability aligns with the broader goal of creating more interpretable AI systems.
- 32
Ethical Considerations: Addressing ethical considerations and ensuring the responsible use of InfoGAN in various applications.
The Power of InfoGAN
InfoGAN is a game-changer in the world of artificial intelligence. It enhances the capabilities of traditional GANs by introducing a way to learn interpretable and disentangled representations. This means InfoGAN can generate more meaningful and varied outputs, making it incredibly useful for tasks like image generation, data augmentation, and even creative applications like art and music.
Understanding the key facts about InfoGAN helps appreciate its potential. From its ability to improve data quality to its role in advancing AI research, InfoGAN stands out as a powerful tool. Its unique approach to learning and generating data opens up new possibilities for innovation.
As AI continues to evolve, InfoGAN will likely play a significant role in shaping the future. Whether you're a researcher, developer, or just curious about AI, knowing about InfoGAN is essential for staying ahead in this exciting field.
Was this page helpful?
Our commitment to delivering trustworthy and engaging content is at the heart of what we do. Each fact on our site is contributed by real users like you, bringing a wealth of diverse insights and information. To ensure the highest standards of accuracy and reliability, our dedicated editors meticulously review each submission. This process guarantees that the facts we share are not only fascinating but also credible. Trust in our commitment to quality and authenticity as you explore and learn with us.