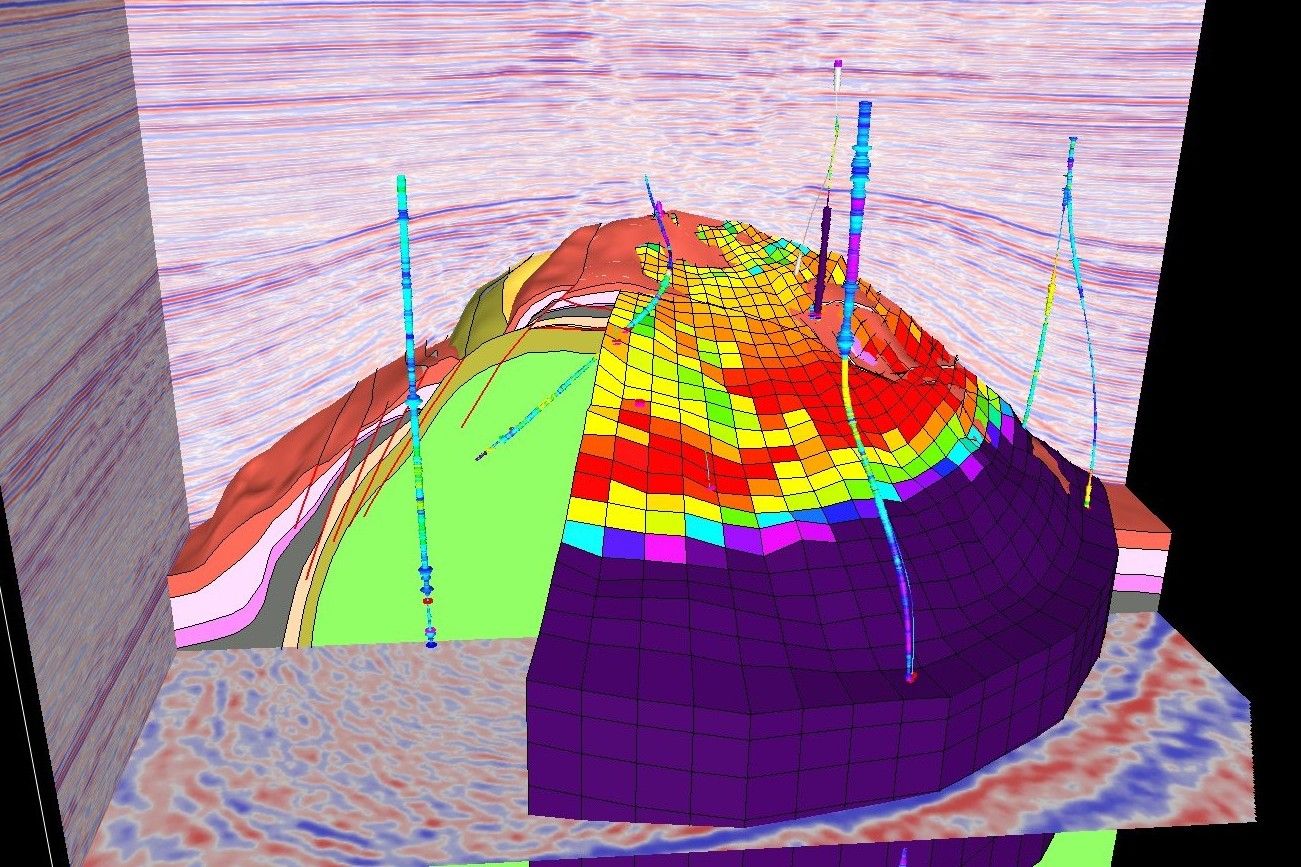
What is residual analysis? Residual analysis is a method used in statistics to check the accuracy of a model. It involves examining the differences between observed and predicted values. These differences, or residuals, help identify if a model fits the data well. By analyzing residuals, one can spot patterns that suggest problems with the model, such as non-linearity or outliers. This technique is crucial for improving models in fields like economics, engineering, and social sciences. Understanding residual analysis can lead to better predictions and more reliable conclusions. Ready to dive into 32 intriguing facts about this essential statistical tool? Let's get started!
What is Residual Analysis?
Residual analysis is a statistical method used to assess the goodness of fit of a model. It involves examining the residuals, which are the differences between observed and predicted values. This technique helps identify any patterns or anomalies that the model may not have captured.
- 01Residuals are the differences between observed values and the values predicted by a model.
- 02Residual analysis helps determine if a model is appropriate for the data.
- 03Patterns in residuals can indicate model inadequacies.
- 04Random residuals suggest a good model fit.
- 05Non-random residuals may indicate a need for model improvement.
Importance of Residual Analysis
Understanding why residual analysis is crucial can help improve the accuracy and reliability of statistical models. It ensures that the model is not just fitting the data but also capturing the underlying patterns.
- 06Detects model bias by showing if residuals are systematically positive or negative.
- 07Improves model accuracy by identifying areas where the model fails.
- 08Ensures reliability by confirming that the model works well for different data sets.
- 09Helps in model selection by comparing residuals from different models.
- 10Identifies outliers that can distort model predictions.
Types of Residuals
Different types of residuals can be used depending on the context and the specific requirements of the analysis. Each type provides unique insights into the model's performance.
- 11Raw residuals are the simplest form, calculated as the difference between observed and predicted values.
- 12Standardized residuals adjust raw residuals by dividing by the standard deviation of the residuals.
- 13Studentized residuals further adjust standardized residuals by considering the influence of each data point.
- 14Pearson residuals are used in generalized linear models and are scaled by the variance of the observed values.
- 15Deviance residuals measure the contribution of each observation to the overall model deviance.
Applications of Residual Analysis
Residual analysis is widely used in various fields to improve model performance and ensure accurate predictions. Its applications range from simple linear regression to complex machine learning models.
- 16Economics uses residual analysis to improve economic models and forecasts.
- 17Engineering applies it to optimize system performance and reliability.
- 18Medicine uses it to refine predictive models for patient outcomes.
- 19Finance employs it to enhance risk assessment models.
- 20Environmental science uses it to improve models predicting climate change effects.
Common Issues in Residual Analysis
Several issues can arise during residual analysis, which can affect the interpretation and reliability of the results. Identifying and addressing these issues is crucial for accurate model evaluation.
- 21Heteroscedasticity occurs when residuals have non-constant variance.
- 22Autocorrelation happens when residuals are correlated with each other.
- 23Non-linearity indicates that the model does not capture the true relationship between variables.
- 24Multicollinearity occurs when independent variables are highly correlated.
- 25Outliers can disproportionately influence the model and residuals.
Techniques to Improve Residual Analysis
Several techniques can be employed to address common issues in residual analysis and improve the overall model fit. These methods help ensure that the residuals are random and the model is reliable.
- 26Transforming variables can help address non-linearity and heteroscedasticity.
- 27Adding interaction terms can capture complex relationships between variables.
- 28Removing outliers can improve model accuracy and residual patterns.
- 29Using robust regression methods can reduce the influence of outliers.
- 30Applying regularization techniques can address multicollinearity.
Tools for Residual Analysis
Various software tools and packages are available to perform residual analysis, making it easier for analysts to evaluate and improve their models.
- 31R offers several packages for residual analysis, such as
lmtest
andcar
. - 32Python provides libraries like
statsmodels
andscikit-learn
for residual analysis.
Wrapping Up Residual Analysis
Residual analysis isn't just for math whizzes. It's a handy tool for anyone wanting to understand data better. By looking at the differences between observed and predicted values, you can spot patterns, outliers, and even errors in your models. This helps improve predictions and make smarter decisions.
Remember, residuals should be randomly scattered around zero. If they aren't, your model might need tweaking. Whether you're working with simple linear regression or more complex models, keeping an eye on residuals can save you from misleading results.
So, next time you're crunching numbers, don't skip the residual analysis. It's like a reality check for your data. Dive in, get your hands dirty, and let those residuals guide you to more accurate insights. Happy analyzing!
Was this page helpful?
Our commitment to delivering trustworthy and engaging content is at the heart of what we do. Each fact on our site is contributed by real users like you, bringing a wealth of diverse insights and information. To ensure the highest standards of accuracy and reliability, our dedicated editors meticulously review each submission. This process guarantees that the facts we share are not only fascinating but also credible. Trust in our commitment to quality and authenticity as you explore and learn with us.